Semi-Label-Text (Index of Posts):
This categoy of tools aims to categorize language text pieces..
This includes:
11 Nov 2020
- authors: Jack Lanchantin, Arshdeep Sekhon, Clint Miller, Yanjun Qi
Abstract
The novel coronavirus SARS-CoV-2, which causes Coronavirus disease 2019 (COVID-19), is a significant threat to worldwide public health. Viruses such as SARS-CoV-2 infect the human body by forming interactions between virus proteins and human proteins that compromise normal human protein-protein interactions (PPI). Current in vivo methods to identify PPIs between a novel virus and humans are slow, costly, and difficult to cover the vast interaction space. We propose a novel deep learning architecture designed for in silico PPI prediction and a transfer learning approach to predict interactions between novel virus proteins and human proteins. We show that our approach outperforms the state-of-the-art methods significantly in predicting Virus–Human protein interactions for SARS-CoV-2, H1N1, and Ebola.
Citations
@article {Lanchantin2020.12.14.422772,
author = {Lanchantin, Jack and Sekhon, Arshdeep and Miller, Clint and Qi, Yanjun},
title = {Transfer Learning with MotifTransformers for Predicting Protein-Protein Interactions Between a Novel Virus and Humans},
elocation-id = {2020.12.14.422772},
year = {2020},
doi = {10.1101/2020.12.14.422772},
publisher = {Cold Spring Harbor Laboratory},
URL = {https://www.biorxiv.org/content/early/2020/12/15/2020.12.14.422772},
eprint = {https://www.biorxiv.org/content/early/2020/12/15/2020.12.14.422772.full.pdf},
journal = {bioRxiv}
}
Having trouble with our tools? Please contact Jack and we’ll help you sort it out.
01 Jun 2020
Title: FastSK: Fast Sequence Analysis with Gapped String Kernels
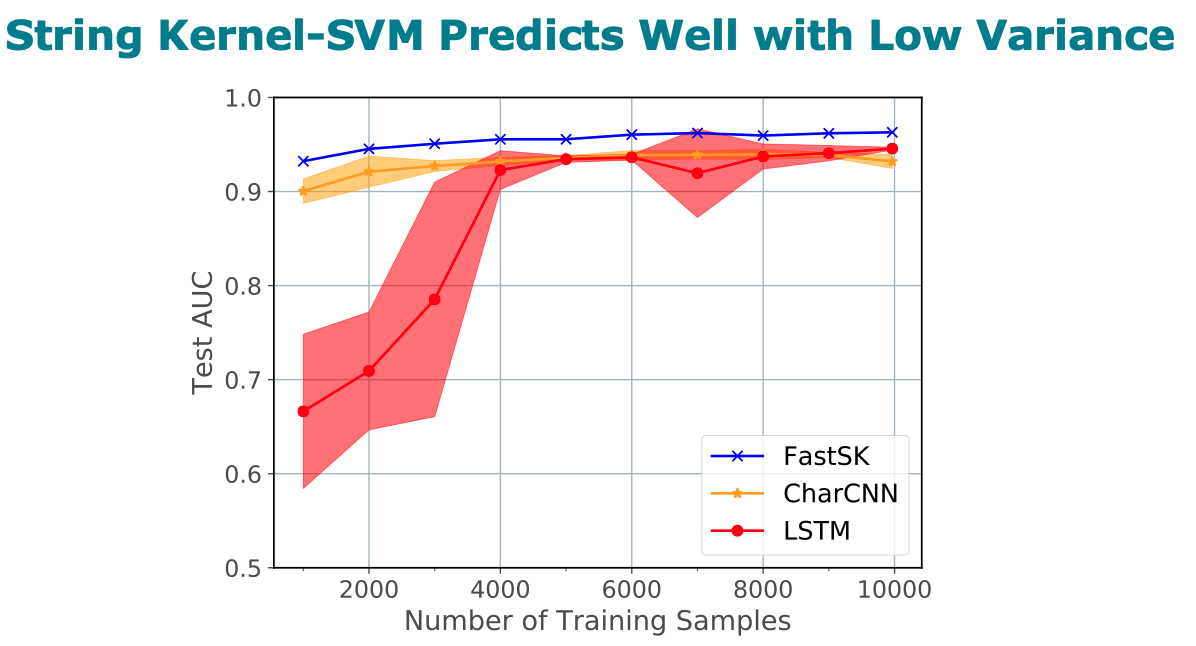
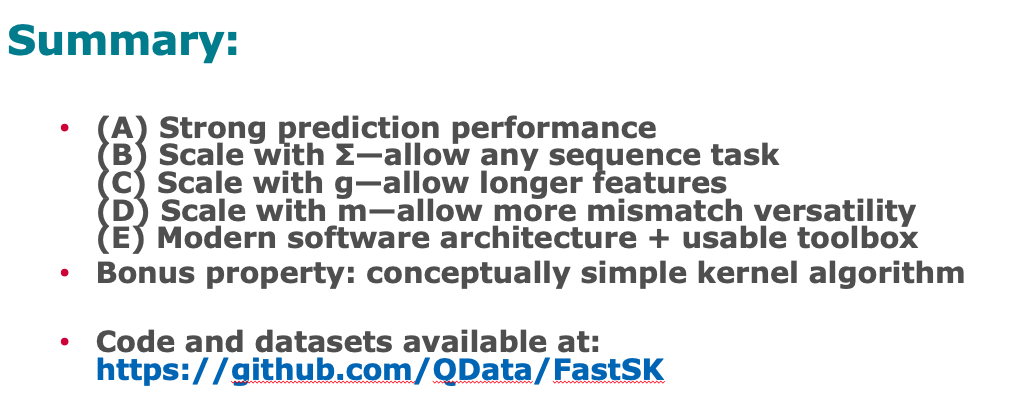
Abstract
Gapped k-mer kernels with Support Vector Machines (gkm-SVMs)
have achieved strong predictive performance on regulatory DNA sequences
on modestly-sized training sets. However, existing gkm-SVM algorithms
suffer from the slow kernel computation time, as they depend
exponentially on the sub-sequence feature-length, number of mismatch
positions, and the task’s alphabet size.
In this work, we introduce a fast and scalable algorithm for
calculating gapped k-mer string kernels. Our method, named FastSK,
uses a simplified kernel formulation that decomposes the kernel
calculation into a set of independent counting operations over the
possible mismatch positions. This simplified decomposition allows us
to devise a fast Monte Carlo approximation that rapidly converges.
FastSK can scale to much greater feature lengths, allows us to
consider more mismatches, and is performant on a variety of sequence
analysis tasks. On 10 DNA transcription factor binding site (TFBS)
prediction datasets, FastSK consistently matches or outperforms the
state-of-the-art gkmSVM-2.0 algorithms in AUC, while achieving
average speedups in kernel computation of 100 times and speedups of
800 times for large feature lengths. We further show that FastSK
outperforms character-level recurrent and convolutional neural
networks across all 10 TFBS tasks. We then extend FastSK to 7
English medical named entity recognition datasets and 10 protein
remote homology detection datasets. FastSK consistently matches or
outperforms these baselines.
Our algorithm is available as a Python package and as C++ source code.
(Available for download at https://github.com/Qdata/FastSK/.
Install with the command make or pip install)
Citations
@article{10.1093/bioinformatics/btaa817,
author = {Blakely, Derrick and Collins, Eamon and Singh, Ritambhara and Norton, Andrew and Lanchantin, Jack and Qi, Yanjun},
title = "{FastSK: fast sequence analysis with gapped string kernels}",
journal = {Bioinformatics},
volume = {36},
number = {Supplement_2},
pages = {i857-i865},
year = {2020},
month = {12},
issn = {1367-4803},
doi = {10.1093/bioinformatics/btaa817},
url = {https://doi.org/10.1093/bioinformatics/btaa817},
eprint = {https://academic.oup.com/bioinformatics/article-pdf/36/Supplement\_2/i857/35337038/btaa817.pdf},
}
Having trouble with our tools? Please contact Yanjun Qi and we’ll help you sort it out.
01 Oct 2013
- authors: Yanjun Qi, Sujatha Das, Ronan Collobert, Jason Weston
Supplementary Here
Abstract
In this paper we introduce a deep neural network architecture to perform information extraction on character-based sequences,
e.g. named-entity recognition on Chinese text or secondary-structure detection on protein sequences. With a task-independent architecture, the
deep network relies only on simple character-based features, which obviates the need for task-specific feature engineering. The proposed discriminative framework includes three important strategies, (1) a deep
learning module mapping characters to vector representations is included
to capture the semantic relationship between characters; (2) abundant
online sequences (unlabeled) are utilized to improve the vector representation through semi-supervised learning; and (3) the constraints of
spatial dependency among output labels are modeled explicitly in the
deep architecture. The experiments on four benchmark datasets have
demonstrated that, the proposed architecture consistently leads to the
state-of-the-art performance.
Citations
@inproceedings{qi2014deep,
title={Deep learning for character-based information extraction},
author={Qi, Yanjun and Das, Sujatha G and Collobert, Ronan and Weston, Jason},
booktitle={European Conference on Information Retrieval},
pages={668--674},
year={2014},
organization={Springer}
}
Having trouble with our tools? Please contact Yanjun Qi and we’ll help you sort it out.
01 Mar 2010
Title: Systems and methods for semi-supervised relationship extraction
- authors: Qi, Yanjun and Bai, Bing and Ning, Xia and Kuksa, Pavel
- PDF
-
Talk: Slide
- Abstract
Bio-relation extraction (bRE), an important goal in bio-text mining, involves subtasks identifying relationships between bio-entities in text at multiple levels, e.g., at the article, sentence or relation level. A key limitation of current bRE systems is that they are restricted by the availability of annotated corpora. In this work we introduce a semi-supervised approach that can tackle multi-level bRE via string comparisons with mismatches in the string kernel framework. Our string kernel implements an abstraction step, which groups similar words to generate more abstract entities, which can be learnt with unlabeled data. Specifically, two unsupervised models are proposed to capture contextual (local or global) semantic similarities between words from a large unannotated corpus. This Abstraction-augmented String Kernel (ASK) allows for better generalization of patterns learned from annotated data and provides a unified framework for solving bRE with multiple degrees
of detail. ASK shows effective improvements over classic string kernels
on four datasets and achieves state-of-the-art bRE performance without
the need for complex linguistic features.
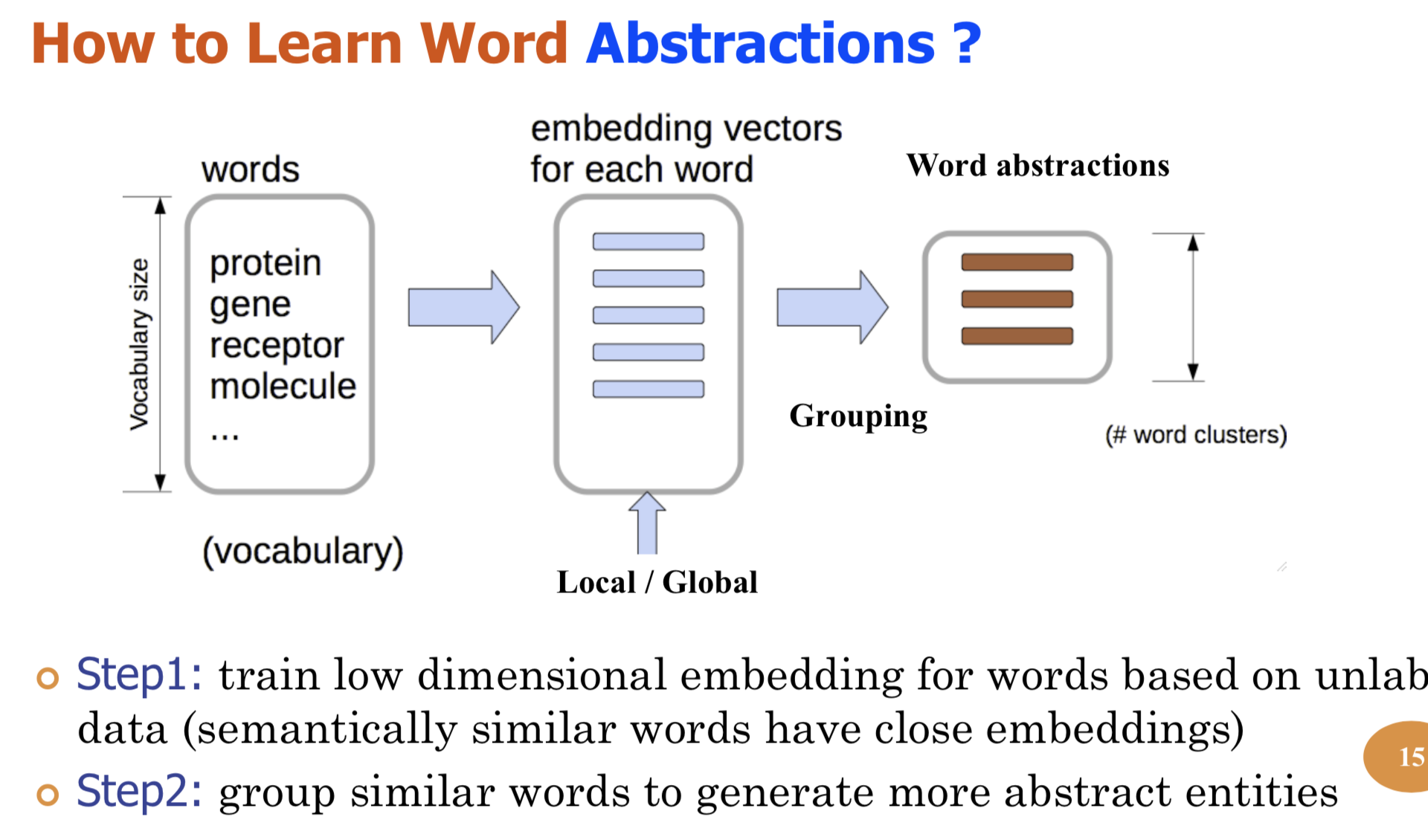
-
PDF
- Talk: Slide
-
URL More
- Abstract
Extracting semantic relations between entities is an important step towards automatic text understanding. In this paper, we propose a novel Semi-supervised Convolution Graph Kernel (SCGK) method for semantic Relation Extraction (RE) from natural language. By encoding English sentences as dependence graphs among words, SCGK computes kernels (similarities) between sentences using a convolution strategy, i.e., calculating similarities over all possible short single paths from two dependence graphs. Furthermore, SCGK adds three semi-supervised strategies in the kernel calculation to incorporate soft-matches between (1) words, (2) grammatical dependencies, and (3) entire sentences, respectively. From a large unannotated corpus, these semi-supervision steps learn to capture contextual semantic patterns of elements in natural sentences, which therefore alleviate the lack of annotated examples in most RE corpora. Through convolutions and multi-level semi-supervisions, SCGK provides a powerful model to encode both syntactic and semantic evidence existing in natural English sentences, which effectively recovers the target relational patterns of interest. We perform extensive experiments on five RE benchmark datasets which aim to identify interaction relations from biomedical literature. Our results demonstrate that SCGK achieves the state-of-the-art performance on the task of semantic relation extraction.
Paper3: Semi-Supervised Bio-Named Entity Recognition with Word-Codebook Learning
- Pavel P. Kuksa, Yanjun Qi,
-
PDF
- Abstract
We describe a novel semi-supervised method called WordCodebook Learning (WCL), and apply it to the task of bionamed entity recognition (bioNER). Typical bioNER systems can be seen as tasks of assigning labels to words in bioliterature text. To improve supervised tagging, WCL learns
a class of word-level feature embeddings to capture word
semantic meanings or word label patterns from a large unlabeled corpus. Words are then clustered according to their
embedding vectors through a vector quantization step, where
each word is assigned into one of the codewords in a codebook. Finally codewords are treated as new word attributes
and are added for entity labeling. Two types of wordcodebook learning are proposed: (1) General WCL, where
an unsupervised method uses contextual semantic similarity of words to learn accurate word representations; (2)
Task-oriented WCL, where for every word a semi-supervised
method learns target-class label patterns from unlabeled
data using supervised signals from trained bioNER model.
Without the need for complex linguistic features, we demonstrate utility of WCL on the BioCreativeII gene name recognition competition data, where WCL yields state-of-the-art
performance and shows great improvements over supervised
baselines and semi-supervised counter peers.
Citations
@INPROCEEDINGS{ecml2010ask,
author = {Pavel P. Kuksa and Yanjun Qi and Bing Bai and Ronan Collobert and
Jason Weston and Vladimir Pavlovic and Xia Ning},
title = {Semi-Supervised Abstraction-Augmented String Kernel for Multi-Level
Bio-Relation Extraction},
booktitle = {ECML},
year = {2010},
note = {Acceptance rate: 106/658 (16%)},
bib2html_pubtype = {Refereed Conference},
}
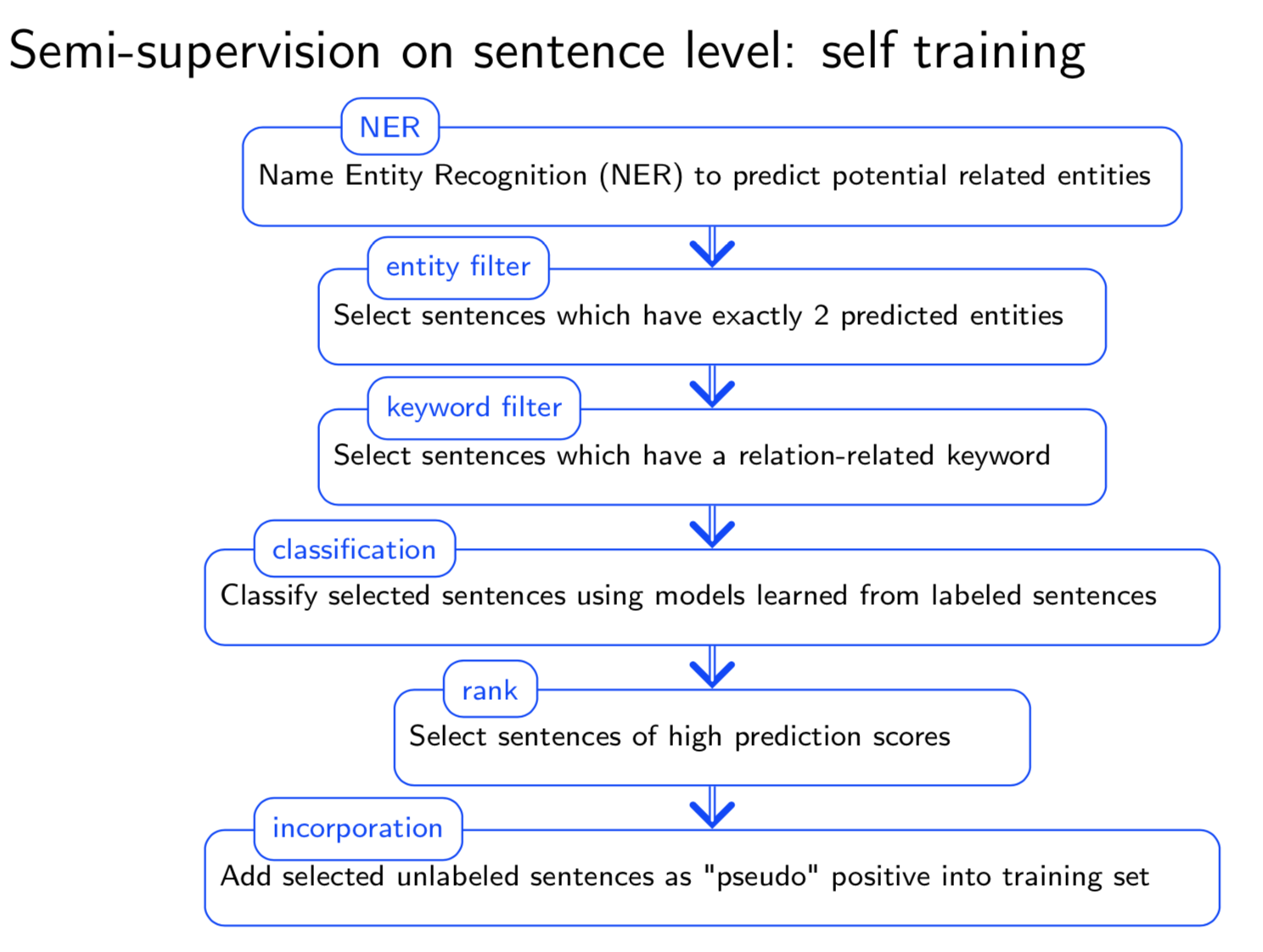
Having trouble with our tools? Please contact Yanjun Qi and we’ll help you sort it out.
01 Oct 2009
Title: Semi-Supervised Sequence Labeling with Self-Learned Feature
- authors: Yanjun
Qi,
Pavel
P
Kuksa,
Ronan
Collobert, Kunihiko
Sadamasa,
Koray
Kavukcuoglu,
Jason
Weston
Abstract
Typical information extraction (IE) systems can be seen as tasks assigning labels to words in a natural language sequence. The performance is restricted by the availability of labeled words. To tackle this issue, we propose a semi-supervised approach to improve the sequence labeling procedure in IE through a class of algorithms with self-learned features (SLF). A supervised classifier can be trained with annotated text sequences and used to classify each word in a large set of unannotated sentences. By averaging predicted labels over all cases in the unlabeled corpus, SLF training builds class label distribution patterns for each word (or word attribute) in the dictionary and re-trains the current model iteratively adding these distributions as extra word features. Basic SLF models how likely a word could be assigned to target class types. Several extensions are proposed, such as learning words’ class boundary distributions. SLF exhibits robust and scalable behaviour and is easy to tune. We applied this approach on four classical IE tasks: named entity recognition (German and English), part-of-speech tagging (English) and one gene name recognition corpus. Experimental results show effective improvements over the supervised baselines on all tasks. In addition, when compared with the closely related self-training idea, this approach shows favorable advantages.
Citations
@inproceedings{qi2009semi,
title={Semi-supervised sequence labeling with self-learned features},
author={Qi, Yanjun and Kuksa, Pavel and Collobert, Ronan and Sadamasa, Kunihiko and Kavukcuoglu, Koray and Weston, Jason},
booktitle={2009 Ninth IEEE International Conference on Data Mining},
pages={428--437},
year={2009},
organization={IEEE}
}
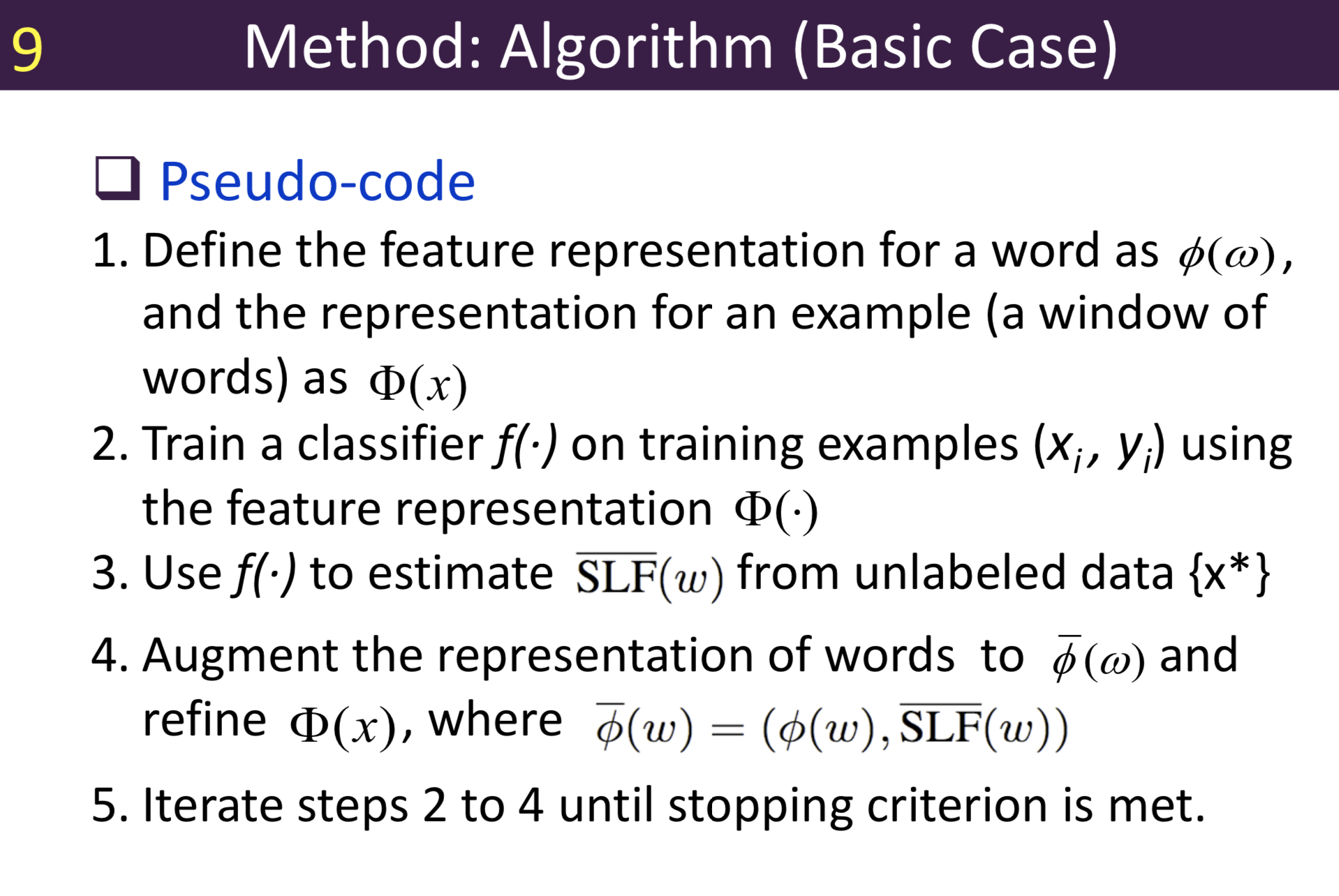
Having trouble with our tools? Please contact Yanjun Qi and we’ll help you sort it out.
01 Feb 2009
Title: Semi-supervised multi-task learning for predicting interactions between HIV-1 and human proteins
- authors: Yanjun Qi, Oznur Tastan, Jaime G. Carbonell, Judith Klein-Seetharaman, Jason Weston
Abstract
-
Motivation: Protein–protein interactions (PPIs) are critical for virtually every biological function. Recently, researchers suggested to use supervised learning for the task of classifying pairs of proteins as interacting or not. However, its performance is largely restricted by the availability of truly interacting proteins (labeled). Meanwhile, there exists a considerable amount of protein pairs where an association appears between two partners, but not enough experimental evidence to support it as a direct interaction (partially labeled).
-
Results: We propose a semi-supervised multi-task framework for predicting PPIs from not only labeled, but also partially labeled reference sets. The basic idea is to perform multi-task learning on a supervised classification task and a semi-supervised auxiliary task. The supervised classifier trains a multi-layer perceptron network for PPI predictions from labeled examples. The semi-supervised auxiliary task shares network layers of the supervised classifier and trains with partially labeled examples. Semi-supervision could be utilized in multiple ways. We tried three approaches in this article, (i) classification (to distinguish partial positives with negatives); (ii) ranking (to rate partial positive more likely than negatives); (iii) embedding (to make data clusters get similar labels). We applied this framework to improve the identification of interacting pairs between HIV-1 and human proteins. Our method improved upon the state-of-the-art method for this task indicating the benefits of semi-supervised multi-task learning using auxiliary information.
Citations
@article{qi2010semi,
title={Semi-supervised multi-task learning for predicting interactions between HIV-1 and human proteins},
author={Qi, Yanjun and Tastan, Oznur and Carbonell, Jaime G and Klein-Seetharaman, Judith and Weston, Jason},
journal={Bioinformatics},
volume={26},
number={18},
pages={i645--i652},
year={2010},
publisher={Oxford University Press}
}
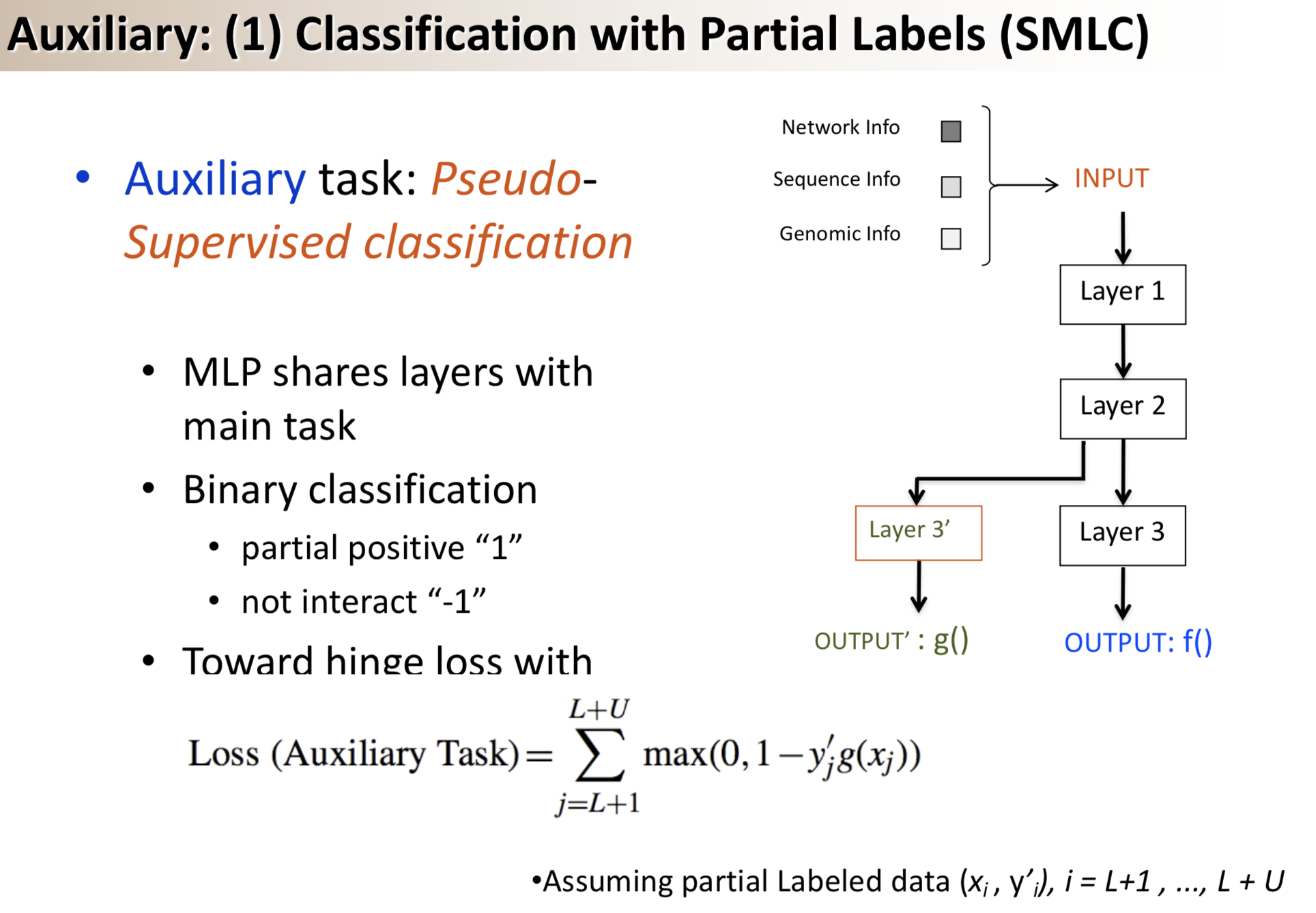
Having trouble with our tools? Please contact Yanjun Qi and we’ll help you sort it out.