1-Evasion
This category of tools aims to automatically assess the robustness of a classifier.
This includes:
12 May 2020
Title: TextAttack: A Framework for Adversarial Attacks in Natural Language Processing
Abstract
TextAttack is a library for generating natural language adversarial examples to fool natural language processing (NLP) models. TextAttack builds attacks from four components: a search method, goal function, transformation, and a set of constraints. Researchers can use these components to easily assemble new attacks. Individual components can be isolated and compared for easier ablation studies. TextAttack currently supports attacks on models trained for text classification and entailment across a variety of datasets. Additionally, TextAttack’s modular design makes it easily extensible to new NLP tasks, models, and attack strategies. TextAttack code and tutorials are available at this https URL.
It is a Python framework for adversarial attacks, data augmentation, and model training in NLP.
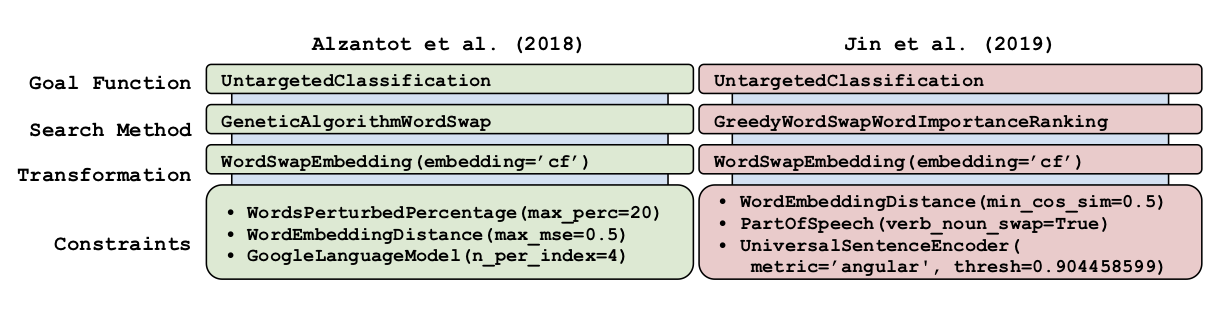
Citations
@misc{morris2020textattack,
title={TextAttack: A Framework for Adversarial Attacks in Natural Language Processing},
author={John X. Morris and Eli Lifland and Jin Yong Yoo and Yanjun Qi},
year={2020},
eprint={2005.05909},
archivePrefix={arXiv},
primaryClass={cs.CL}
}
Having trouble with our tools? Please contact DrQ and we’ll help you sort it out.
12 Jan 2018
Title: Black-box Generation of Adversarial Text Sequences to Fool Deep Learning Classifiers
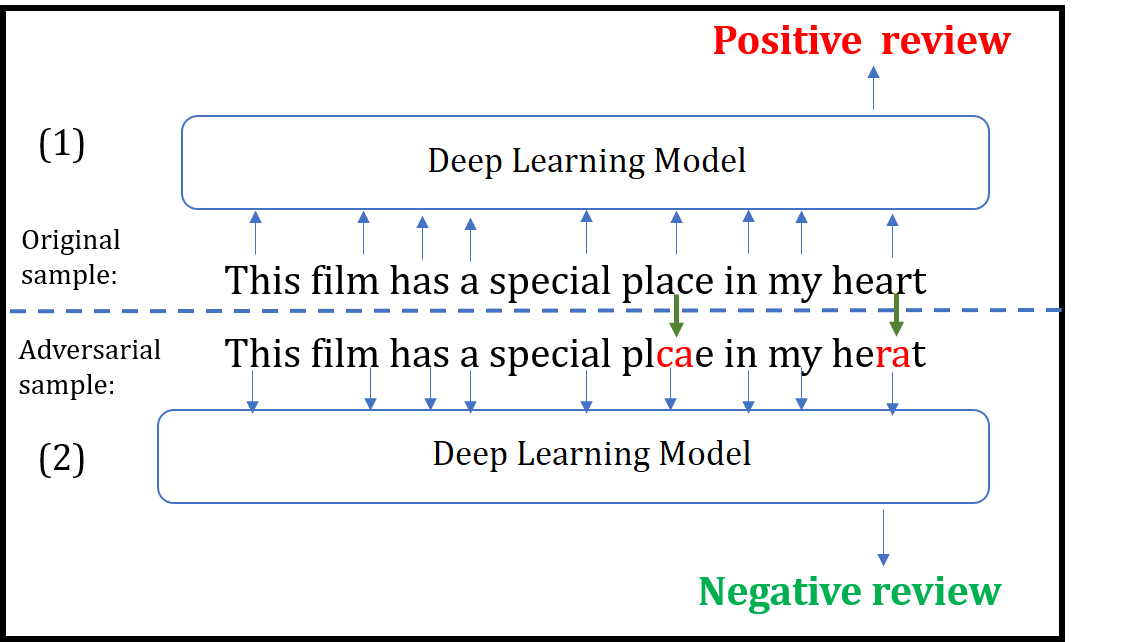
TalkSlide: URL
Published @ 2018 IEEE Security and Privacy Workshops (SPW),
co-located with the 39th IEEE Symposium on Security and Privacy.
Abstract
Although various techniques have been proposed to generate adversarial samples for white-box attacks on text, little attention has been paid to a black-box attack, which is a more realistic scenario. In this paper, we present a novel algorithm, DeepWordBug, to effectively generate small text perturbations in a black-box setting that forces a deep-learning classifier to misclassify a text input. We develop novel scoring strategies to find the most important words to modify such that the deep classifier makes a wrong prediction. Simple character-level transformations are applied to the highest-ranked words in order to minimize the edit distance of the perturbation. We evaluated DeepWordBug on two real-world text datasets: Enron spam emails and IMDB movie reviews. Our experimental results indicate that DeepWordBug can reduce the classification accuracy from 99% to around 40% on Enron data and from 87% to about 26% on IMDB. Also, our experimental results strongly demonstrate that the generated adversarial sequences from a deep-learning model can similarly evade other deep models.
We build an interactive extension to visualize DeepWordbug:
- Interactive Live Demo @ ULR
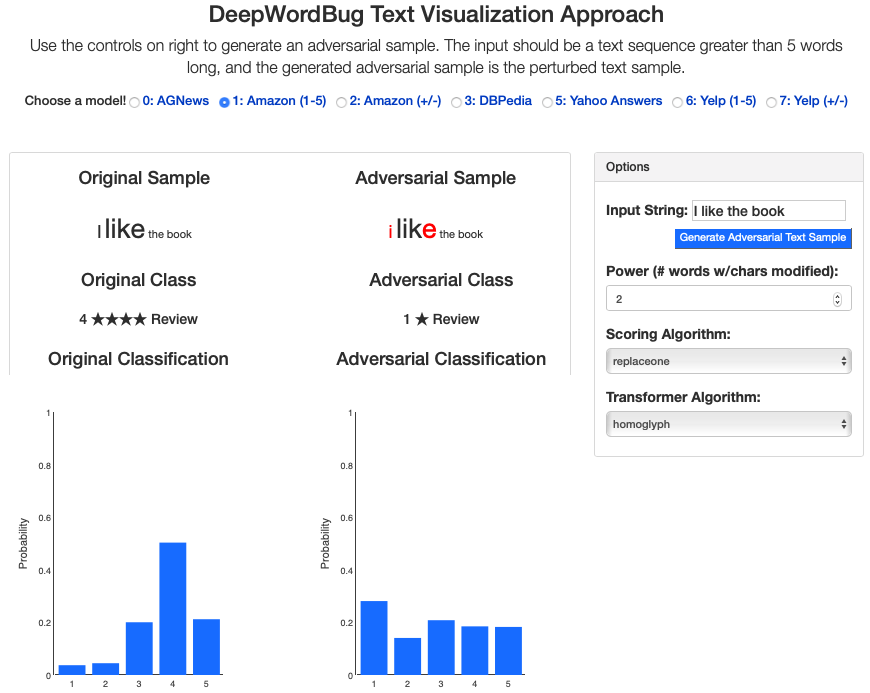
Citations
@INPROCEEDINGS{JiDeepWordBug18,
author={J. Gao and J. Lanchantin and M. L. Soffa and Y. Qi},
booktitle={2018 IEEE Security and Privacy Workshops (SPW)},
title={Black-Box Generation of Adversarial Text Sequences to Evade Deep Learning Classifiers},
year={2018},
pages={50-56},
keywords={learning (artificial intelligence);pattern classification;program debugging;text analysis;deep learning classifiers;character-level transformations;IMDB movie reviews;Enron spam emails;real-world text datasets;scoring strategies;text input;text perturbations;DeepWordBug;black-box attack;adversarial text sequences;black-box generation;Perturbation methods;Machine learning;Task analysis;Recurrent neural networks;Prediction algorithms;Sentiment analysis;adversarial samples;black box attack;text classification;misclassification;word embedding;deep learning},
doi={10.1109/SPW.2018.00016},
month={May},}
Having trouble with our tools? Please contact me and we’ll help you sort it out.
01 Jun 2017
Paper: Automatically Evading Classifiers,
A Case Study on PDF Malware Classifiers NDSS16
More information is provided by EvadeML.org
By using evolutionary techniques to simulate an adversary’s efforts to evade that classifier
Abstract
Machine learning is widely used to develop classifiers for security tasks. However, the robustness of these methods
against motivated adversaries is uncertain. In this work, we
propose a generic method to evaluate the robustness of classifiers
under attack. The key idea is to stochastically manipulate a
malicious sample to find a variant that preserves the malicious
behavior but is classified as benign by the classifier. We present
a general approach to search for evasive variants and report on
results from experiments using our techniques against two PDF
malware classifiers, PDFrate and Hidost. Our method is able to
automatically find evasive variants for both classifiers for all of
the 500 malicious seeds in our study. Our results suggest a general
method for evaluating classifiers used in security applications, and
raise serious doubts about the effectiveness of classifiers based
on superficial features in the presence of adversaries.
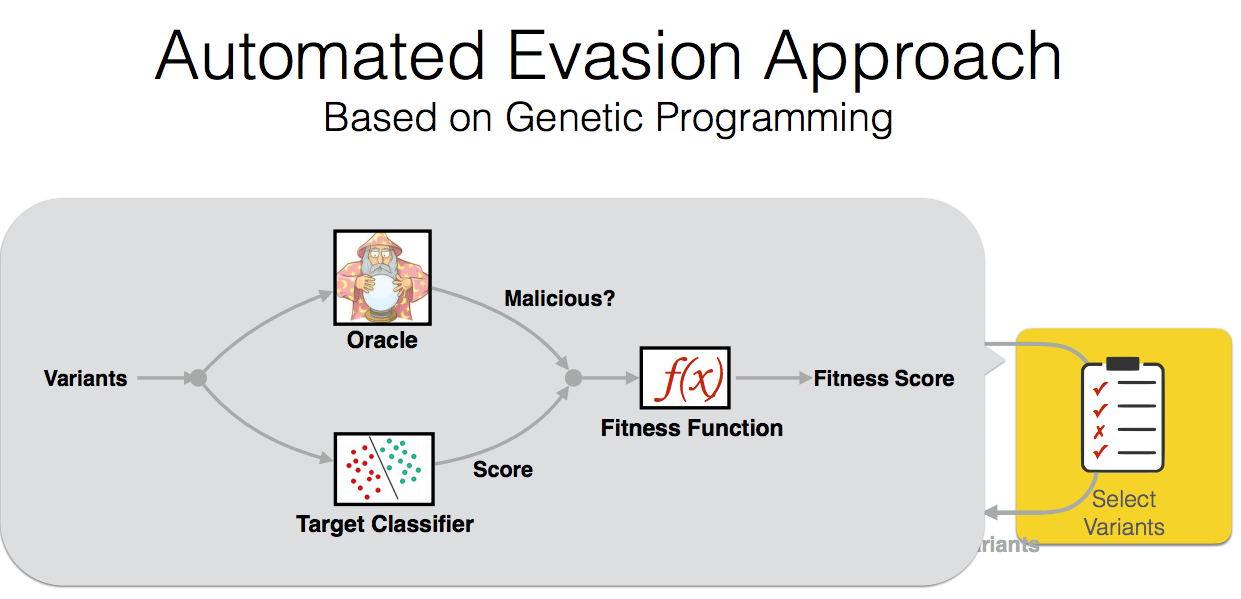
Citations
@inproceedings{xu2016automatically,
title={Automatically evading classifiers},
author={Xu, Weilin and Qi, Yanjun and Evans, David},
booktitle={Proceedings of the 2016 Network and Distributed Systems Symposium},
year={2016}
}
Having troubl with our tools? Please contact Weilin and we’ll help you sort it out.