DeepSequence (Index of Posts):
We create this categoy of deep learning tools to improve representation learning on bio-inputs following a sequential ordering (e.g., signals on genome, epigenome, protein)..
This includes:
-
Transfer Learning with Motif Transformers for Predicting Protein-Protein Interactions Between a Novel Virus and Humans
November 11, 2020
-
Graph Convolutional Networks for Epigenetic State Prediction Using Both Sequence and 3D Genome Data
May 1, 2020
-
DeepDiff- Deep-learning for predicting Differential gene expression from histone modifications
September 7, 2018
-
Best Paper Award for Deep Motif Dashboard
December 11, 2017
-
Prototype Matching Networks for Large-Scale Multi-label Genomic Sequence Classification
December 10, 2017
-
AttentiveChrome-Deep Attention Model to Understand Gene Regulation by Selective Attention on Chromatin
July 30, 2017
-
Memory Matching Networks for Genomic Sequence Classification
June 12, 2017
-
Deep Motif Dashboard- Visualizing and Understanding Genomic Sequences Using Deep Neural Networks
June 11, 2017
-
DeepChrome- deep-learning for predicting gene expression from histone modifications
June 10, 2017
-
MUST-CNN- A Multilayer Shift-and-Stitch Deep Convolutional Architecture for Sequence-based Protein Structure Prediction
June 11, 2015
-
A unified multitask architecture for predicting local protein properties
January 12, 2015
-
Deep Learning for Character-based Information Extraction on Chinese and Protein Sequence
October 1, 2013
-
A unified multitask architecture for predicting local structural properties on proteins
January 12, 2013
-
Systems and methods for semi-supervised relationship extraction
March 1, 2010
-
Semi-supervised multi-task learning Using BioText based Labels to Augument PPI Prediction
February 1, 2009
11 Nov 2020
- authors: Jack Lanchantin, Arshdeep Sekhon, Clint Miller, Yanjun Qi
Abstract
The novel coronavirus SARS-CoV-2, which causes Coronavirus disease 2019 (COVID-19), is a significant threat to worldwide public health. Viruses such as SARS-CoV-2 infect the human body by forming interactions between virus proteins and human proteins that compromise normal human protein-protein interactions (PPI). Current in vivo methods to identify PPIs between a novel virus and humans are slow, costly, and difficult to cover the vast interaction space. We propose a novel deep learning architecture designed for in silico PPI prediction and a transfer learning approach to predict interactions between novel virus proteins and human proteins. We show that our approach outperforms the state-of-the-art methods significantly in predicting Virus–Human protein interactions for SARS-CoV-2, H1N1, and Ebola.
Citations
@article {Lanchantin2020.12.14.422772,
author = {Lanchantin, Jack and Sekhon, Arshdeep and Miller, Clint and Qi, Yanjun},
title = {Transfer Learning with MotifTransformers for Predicting Protein-Protein Interactions Between a Novel Virus and Humans},
elocation-id = {2020.12.14.422772},
year = {2020},
doi = {10.1101/2020.12.14.422772},
publisher = {Cold Spring Harbor Laboratory},
URL = {https://www.biorxiv.org/content/early/2020/12/15/2020.12.14.422772},
eprint = {https://www.biorxiv.org/content/early/2020/12/15/2020.12.14.422772.full.pdf},
journal = {bioRxiv}
}
Having trouble with our tools? Please contact Jack and we’ll help you sort it out.
01 May 2020
Title: Graph Convolutional Networks for Epigenetic State Prediction Using Both Sequence and 3D Genome Data
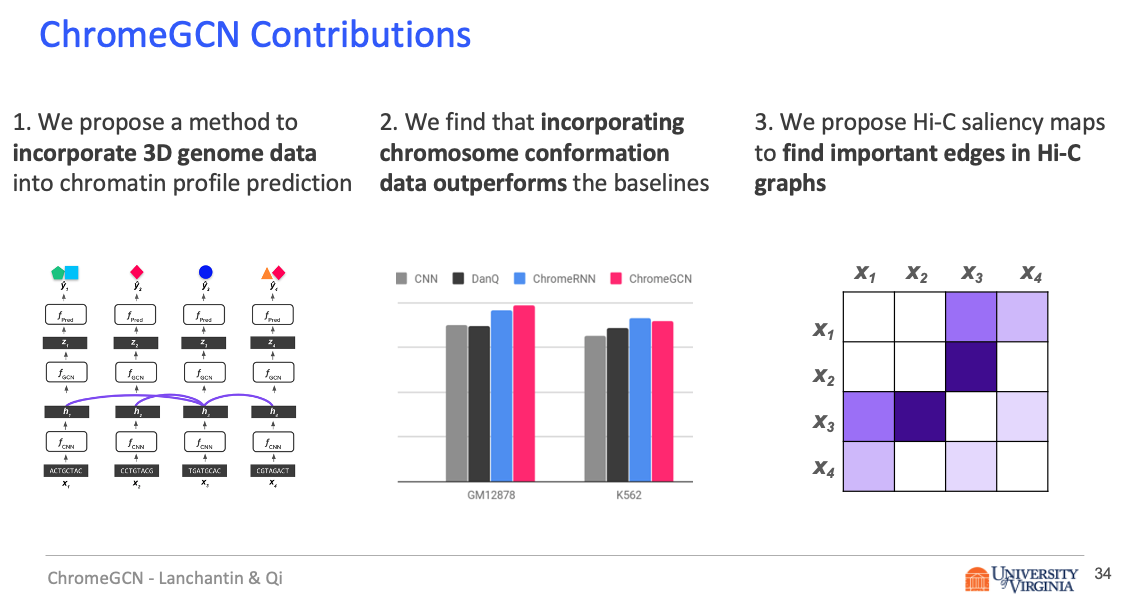
Abstract
Motivation
Predictive models of DNA chromatin profile (i.e. epigenetic state), such as transcription factor binding, are essential for understanding regulatory processes and developing gene therapies. It is known that the 3D genome, or spatial structure of DNA, is highly influential in the chromatin profile. Deep neural networks have achieved state of the art performance on chromatin profile prediction by using short windows of DNA sequences independently. These methods, however, ignore the long-range dependencies when predicting the chromatin profiles because modeling the 3D genome is challenging.
Results
In this work, we introduce ChromeGCN, a graph convolutional network for chromatin profile prediction by fusing both local sequence and long-range 3D genome information. By incorporating the 3D genome, we relax the independent and identically distributed assumption of local windows for a better representation of DNA. ChromeGCN explicitly incorporates known long-range interactions into the modeling, allowing us to identify and interpret those important long-range dependencies in influencing chromatin profiles. We show experimentally that by fusing sequential and 3D genome data using ChromeGCN, we get a significant improvement over the state-of-the-art deep learning methods as indicated by three metrics. Importantly, we show that ChromeGCN is particularly useful for identifying epigenetic effects in those DNA windows that have a high degree of interactions with other DNA windows.
Citations
@article{10.1093/bioinformatics/btaa793,
author = {Lanchantin, Jack and Qi, Yanjun},
title = "{Graph convolutional networks for epigenetic state prediction using both sequence and 3D genome data}",
journal = {Bioinformatics},
volume = {36},
number = {Supplement_2},
pages = {i659-i667},
year = {2020},
month = {12},
issn = {1367-4803},
doi = {10.1093/bioinformatics/btaa793},
url = {https://doi.org/10.1093/bioinformatics/btaa793},
eprint = {https://academic.oup.com/bioinformatics/article-pdf/36/Supplement\_2/i659/35336695/btaa793.pdf},
}
Having trouble with our tools? Please contact Jack and we’ll help you sort it out.
07 Sep 2018
Paper:
Abstract:
Computational methods that predict differential gene expression from histone modification signals are highly desirable for understanding how histone modifications control the functional heterogeneity of cells through influencing differential gene regulation. Recent studies either failed to capture combinatorial effects on differential prediction or primarily only focused on cell type-specific analysis. In this paper, we develop a novel attention-based deep learning architecture, DeepDiff, that provides a unified and end-to-end solution to model and to interpret how dependencies among histone modifications control the differential patterns of gene regulation. DeepDiff uses a hierarchy of multiple Long short-term memory (LSTM) modules to encode the spatial structure of input signals and to model how various histone modifications cooperate automatically. We introduce and train two levels of attention jointly with the target prediction, enabling DeepDiff to attend differentially to relevant modifications and to locate important genome positions for each modification. Additionally, DeepDiff introduces a novel deep-learning based multi-task formulation to use the cell-type-specific gene expression predictions as auxiliary tasks, encouraging richer feature embeddings in our primary task of differential expression prediction. Using data from Roadmap Epigenomics Project (REMC) for ten different pairs of cell types, we show that DeepDiff significantly outperforms the state-of-the-art baselines for differential gene expression prediction. The learned attention weights are validated by observations from previous studies about how epigenetic mechanisms connect to differential gene expression. Codes and results are available at deepchrome.org
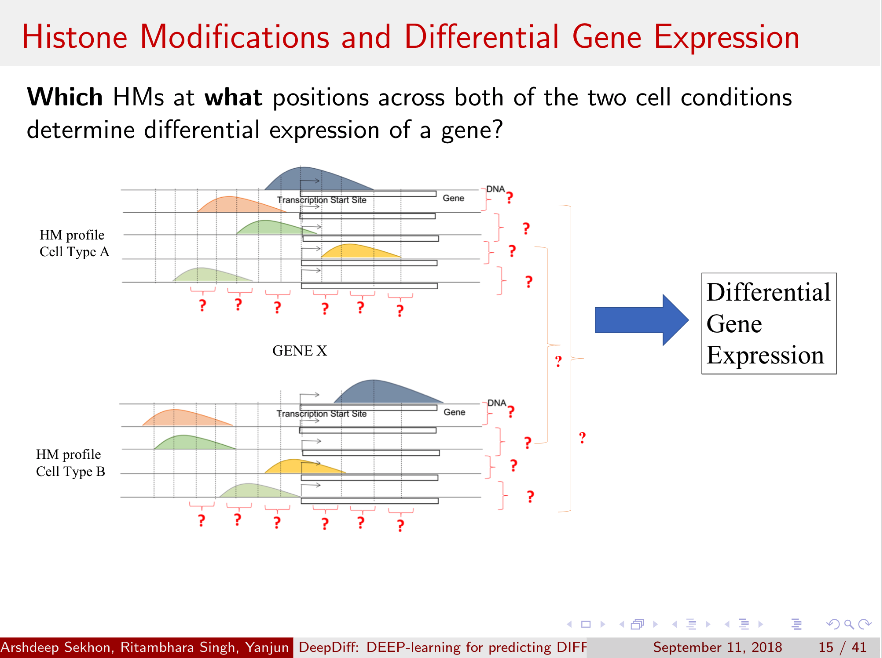
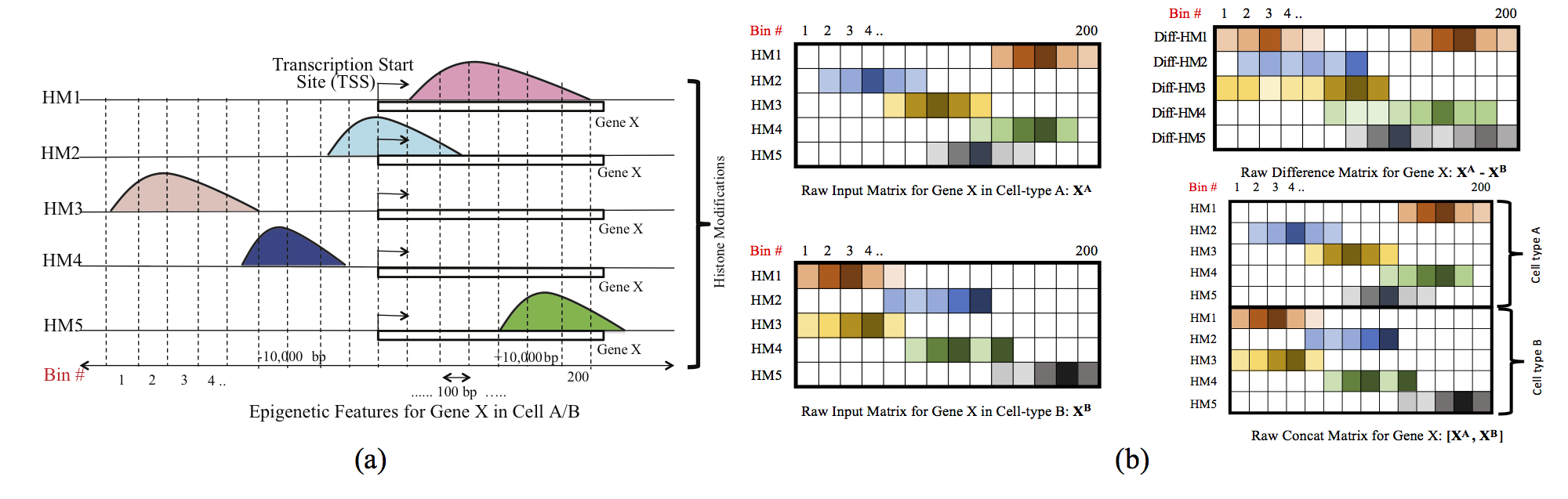
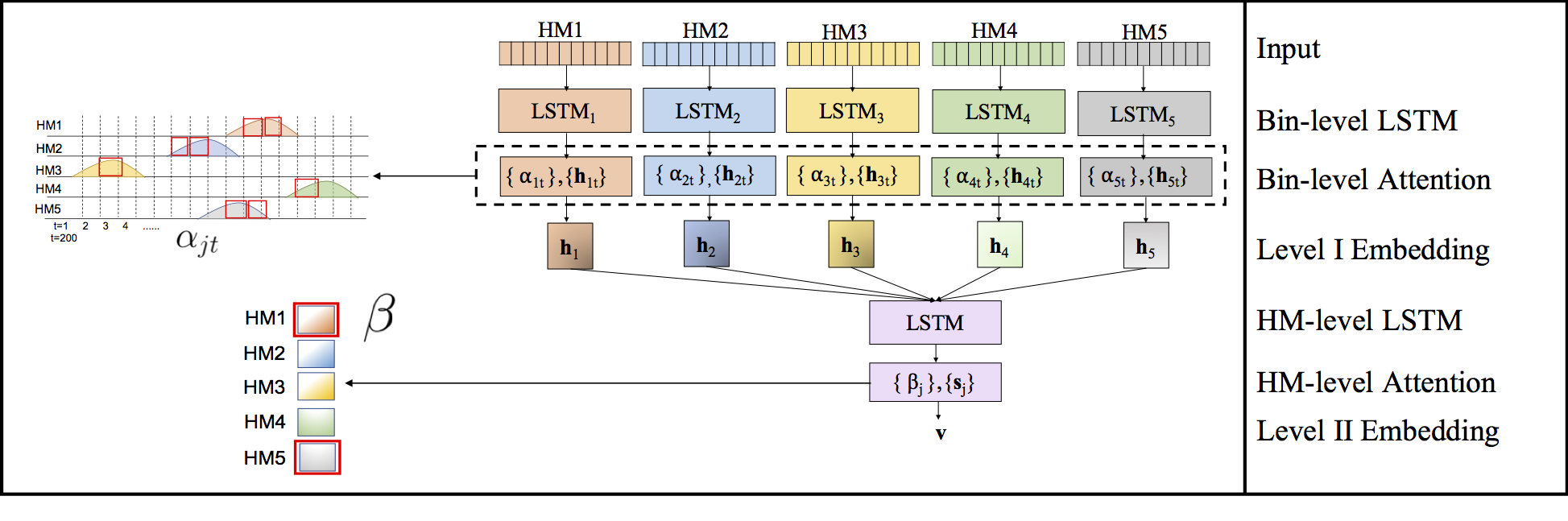
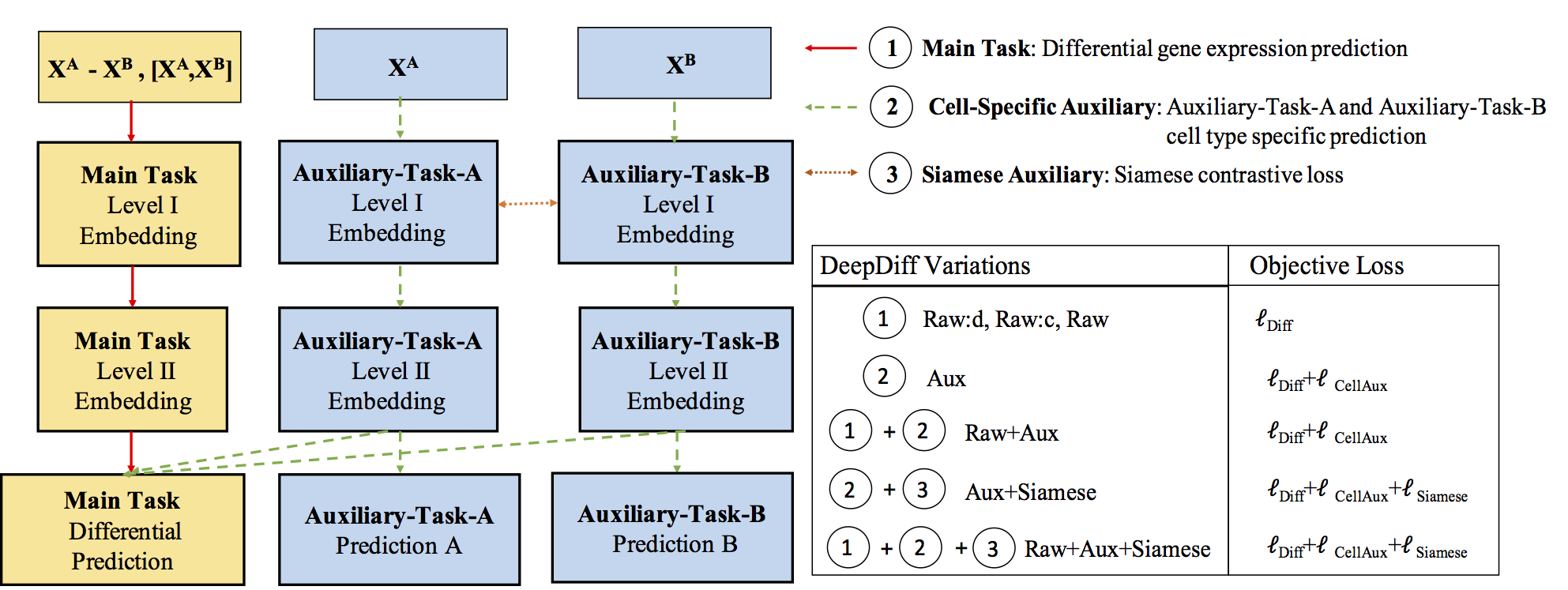
Citations
@article{ArDeepDiff18,
author = {Sekhon, Arshdeep and Singh, Ritambhara and Qi, Yanjun},
title = {DeepDiff: DEEP-learning for predicting DIFFerential gene expression from histone modifications},
journal = {Bioinformatics},
volume = {34},
number = {17},
pages = {i891-i900},
year = {2018},
doi = {10.1093/bioinformatics/bty612},
URL = {http://dx.doi.org/10.1093/bioinformatics/bty612},
eprint = {/oup/backfile/content_public/journal/bioinformatics/34/17/10.1093_bioinformatics_bty612/2/bty612.pdf}
}
Having trouble with our tools? Please contact Arsh and we’ll help you sort it out.
11 Dec 2017
Jack’s DeepMotif paper (Deep Motif Dashboard: Visualizing and Understanding Genomic Sequences Using Deep Neural Networks ) have received the “best paper award“ at NIPS17 workshop for Transparent and interpretable Machine Learning in Safety Critical Environments. Big congratulations!!!
10 Dec 2017
Prototype Matching Networks : A novel deep learning architecture for Large-Scale Multi-label Genomic Sequence Classification
Abstract
One of the fundamental tasks in understanding genomics is the problem of predicting Transcription Factor Binding Sites (TFBSs). With more than hundreds of Transcription Factors (TFs) as labels, genomic-sequence based TFBS prediction is a challenging multi-label classification task. There are two major biological mechanisms for TF binding: (1) sequence-specific binding patterns on genomes known as “motifs” and (2) interactions among TFs known as co-binding effects. In this paper, we propose a novel deep architecture, the Prototype Matching Network (PMN) to mimic the TF binding mechanisms. Our PMN model automatically extracts prototypes (“motif”-like features) for each TF through a novel prototype-matching loss. Borrowing ideas from few-shot matching models, we use the notion of support set of prototypes and an LSTM to learn how TFs interact and bind to genomic sequences. On a reference TFBS dataset with 2.1 million genomic sequences, PMN significantly outperforms baselines and validates our design choices empirically. To our knowledge, this is the first deep learning architecture that introduces prototype learning and considers TF-TF interactions for large-scale TFBS prediction. Not only is the proposed architecture accurate, but it also models the underlying biology.
Citations
@article{lanchantin2017prototype,
title={Prototype Matching Networks for Large-Scale Multi-label Genomic Sequence Classification},
author={Lanchantin, Jack and Sekhon, Arshdeep and Singh, Ritambhara and Qi, Yanjun},
journal={arXiv preprint arXiv:1710.11238},
year={2017}
}
Having trouble with our tools? Please contact Jack and we’ll help you sort it out.
30 Jul 2017
Paper: @Arxiv + Published at [NIPS2017]
(https://papers.nips.cc/paper/7255-attend-and-predict-understanding-gene-regulation-by-selective-attention-on-chromatin.pdf)
Abstract:
The past decade has seen a revolution in genomic technologies that enable a flood of genome-wide profiling of chromatin marks. Recent literature tried to understand gene regulation by predicting gene expression from large-scale chromatin measurements. Two fundamental challenges exist for such learning tasks: (1) genome-wide chromatin signals are spatially structured, high-dimensional and highly modular; and (2) the core aim is to understand what are the relevant factors and how they work together? Previous studies either failed to model complex dependencies among input signals or relied on separate feature analysis to explain the decisions. This paper presents an attention-based deep learning approach; we call AttentiveChrome, that uses a unified architecture to model and to interpret dependencies among chromatin factors for controlling gene regulation. AttentiveChrome uses a hierarchy of multiple Long short-term memory (LSTM) modules to encode the input signals and to model how various chromatin marks cooperate automatically. AttentiveChrome trains two levels of attention jointly with the target prediction, enabling it to attend differentially to relevant marks and to locate important positions per mark. We evaluate the model across 56 different cell types (tasks) in human. Not only is the proposed architecture more accurate, but its attention scores also provide a better interpretation than state-of-the-art feature visualization methods such as saliency map.
Code and data are shared at www.deepchrome.net
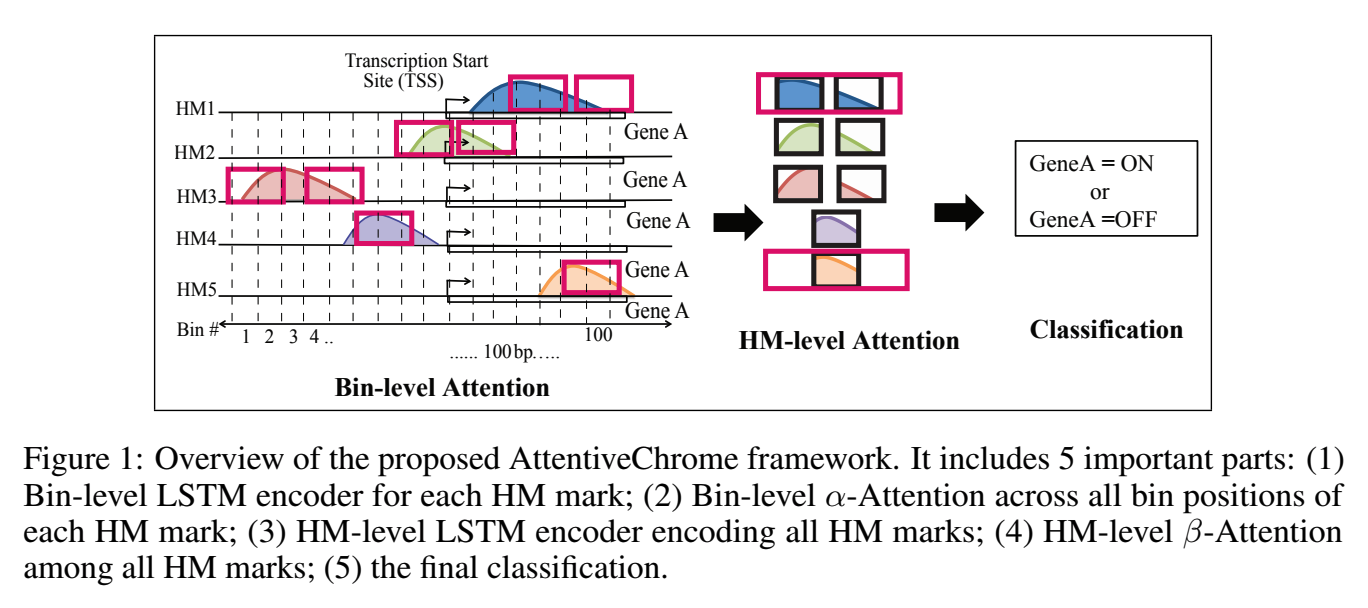
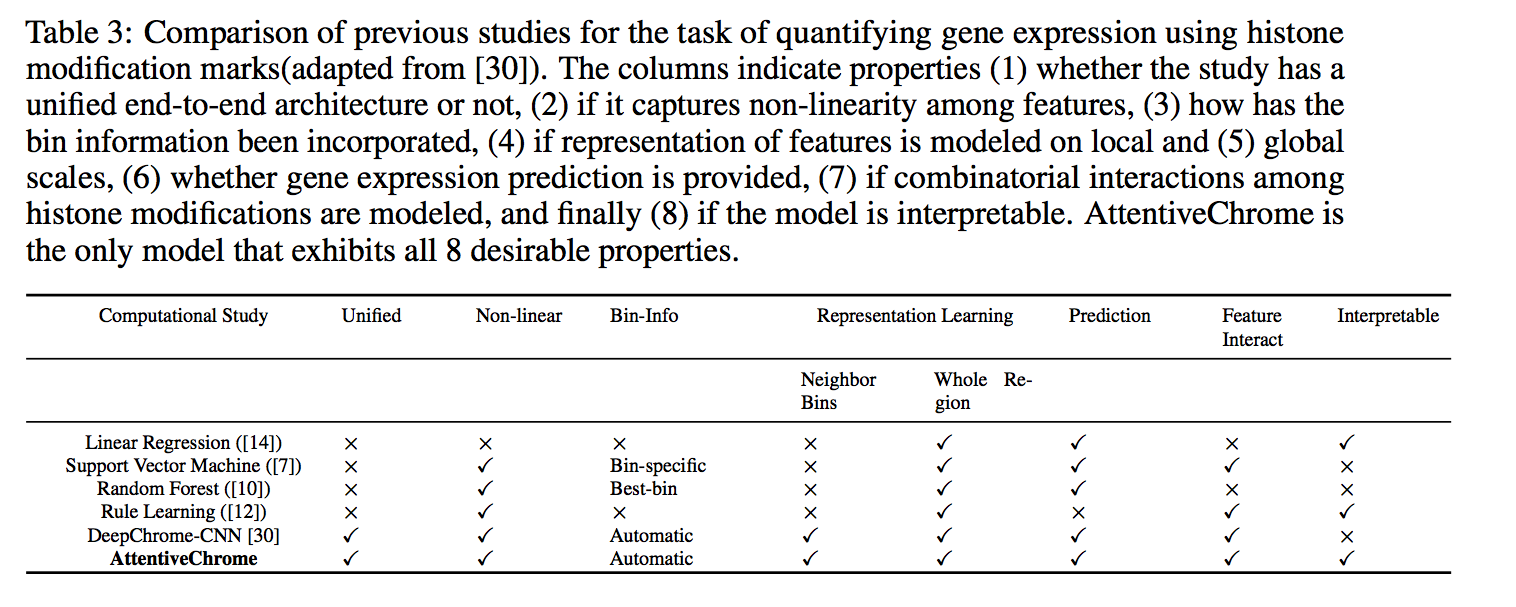
Citations
@inproceedings{singh2017attend,
title={Attend and Predict: Understanding Gene Regulation by Selective Attention on Chromatin},
author={Singh, Ritambhara and Lanchantin, Jack and Sekhon, Arshdeep and Qi, Yanjun},
booktitle={Advances in Neural Information Processing Systems},
pages={6769--6779},
year={2017}
}
Having trouble with our tools? Please contact Rita and we’ll help you sort it out.
12 Jun 2017
Abstract
When analyzing the genome, researchers have discovered that proteins bind to DNA based on certain patterns of the DNA sequence known as “motifs”. However, it is difficult to manually construct motifs due to their complexity. Recently, externally learned memory models have proven to be effective methods for reasoning over inputs and supporting sets. In this work, we present memory matching networks (MMN) for classifying DNA sequences as protein binding sites. Our model learns a memory bank of encoded motifs, which are dynamic memory modules, and then matches a new test sequence to each of the motifs to classify the sequence as a binding or nonbinding site.
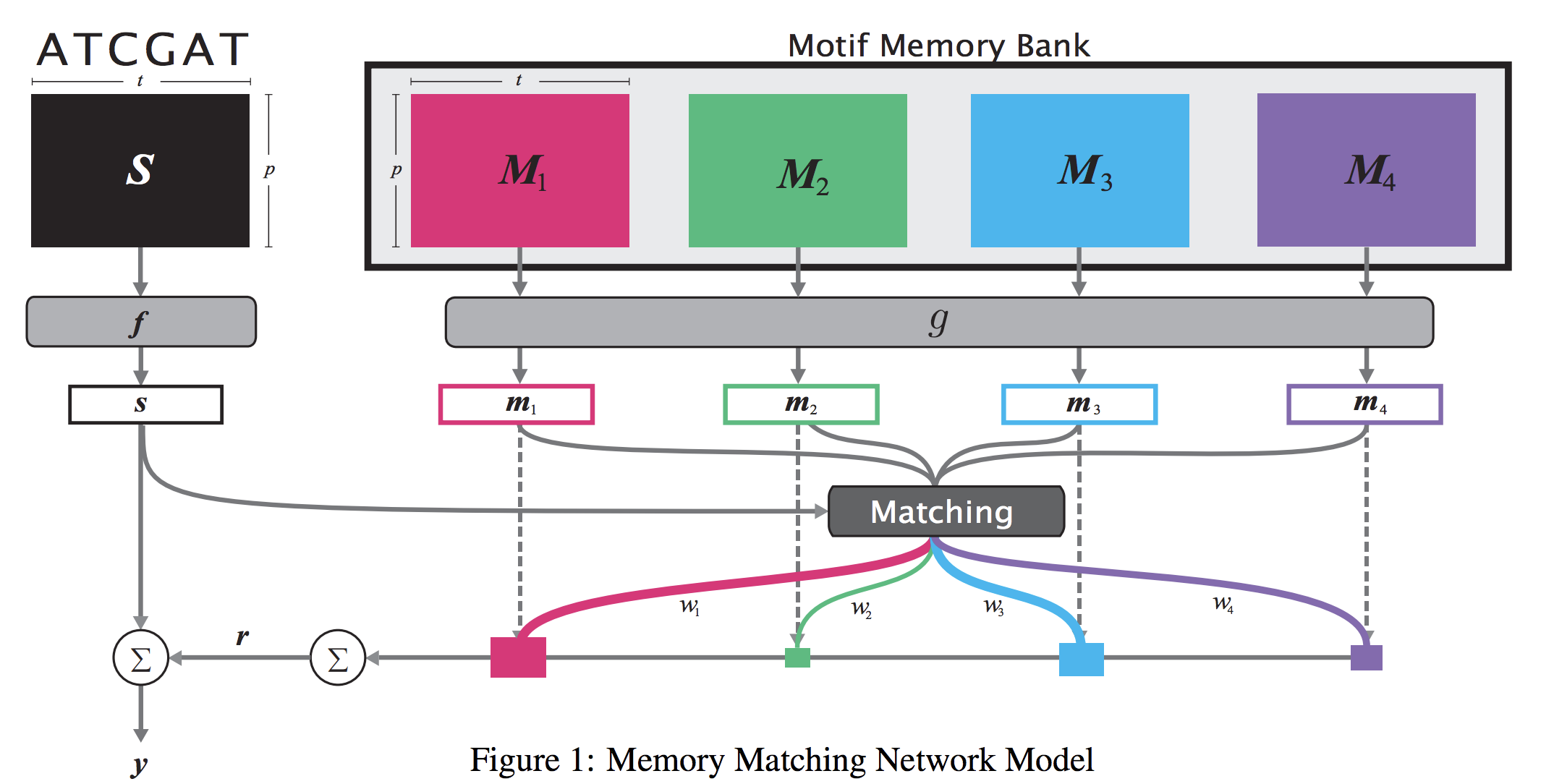
Citations
@article{lanchantin2017memory,
title={Memory Matching Networks for Genomic Sequence Classification},
author={Lanchantin, Jack and Singh, Ritambhara and Qi, Yanjun},
journal={arXiv preprint arXiv:1702.06760},
year={2017}
}
Having trouble with our tools? Please contact Jack and we’ll help you sort it out.
11 Jun 2017
Abstract:
Deep neural network (DNN) models have recently obtained state-of-the-art prediction accuracy for the transcription factor binding (TFBS) site classification task. However, it remains unclear how these approaches identify meaningful DNA sequence signals and give insights as to why TFs bind to certain locations. In this paper, we propose a toolkit called the Deep Motif Dashboard (DeMo Dashboard) which provides a suite of visualization strategies to extract motifs, or sequence patterns from deep neural network models for TFBS classification. We demonstrate how to visualize and understand three important DNN models: convolutional, recurrent, and convolutional-recurrent networks. Our first visualization method is finding a test sequence’s saliency map which uses first-order derivatives to describe the importance of each nucleotide in making the final prediction. Second, considering recurrent models make predictions in a temporal manner (from one end of a TFBS sequence to the other), we introduce temporal output scores, indicating the prediction score of a model over time for a sequential input. Lastly, a class-specific visualization strategy finds the optimal input sequence for a given TFBS positive class via stochastic gradient optimization. Our experimental results indicate that a convolutional-recurrent architecture performs the best among the three architectures. The visualization techniques indicate that CNN-RNN makes predictions by modeling both motifs as well as dependencies among them.
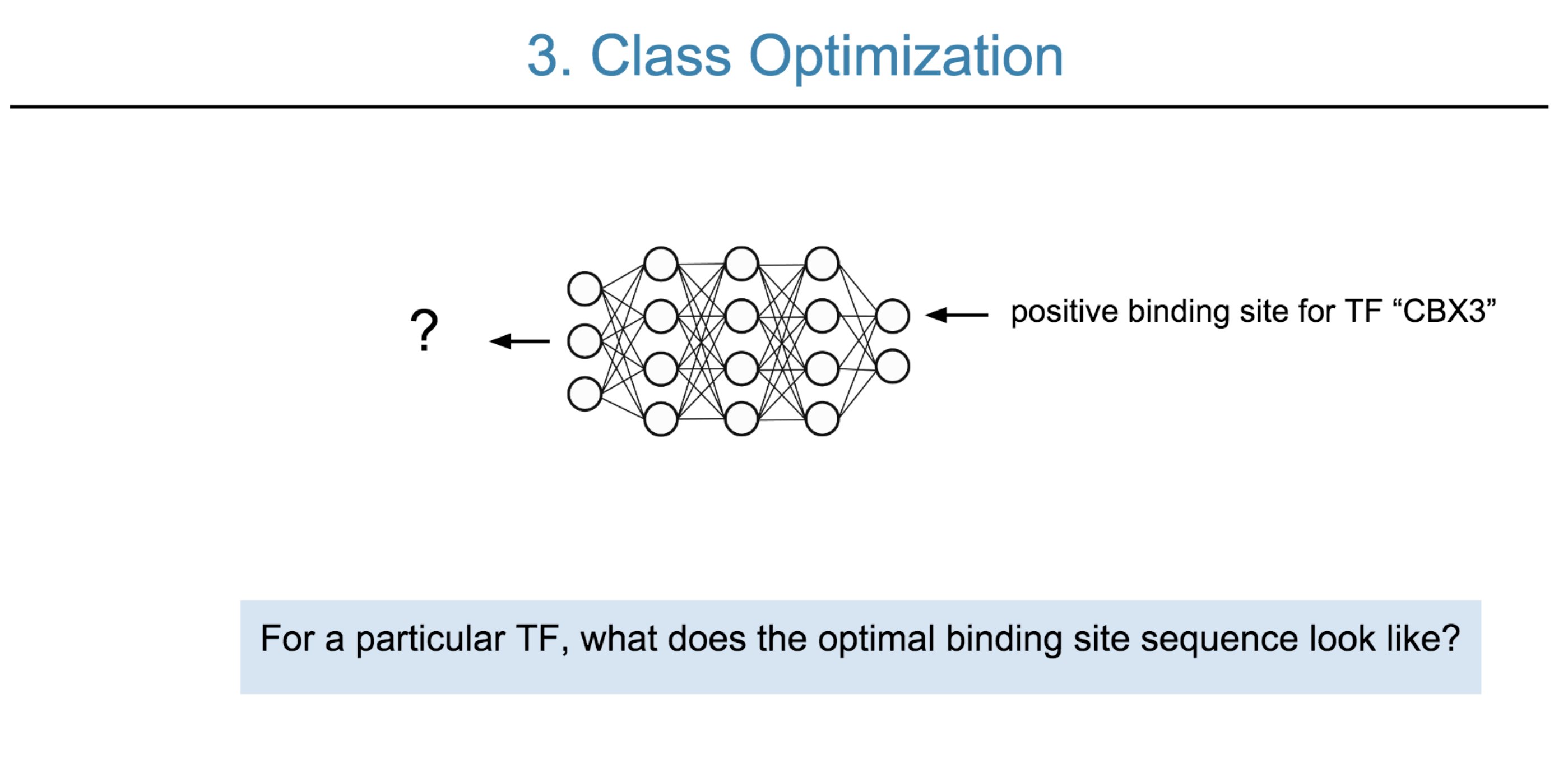
Citations
@inproceedings{lanchantin2017deep,
title={Deep motif dashboard: Visualizing and understanding genomic sequences using deep neural networks},
author={Lanchantin, Jack and Singh, Ritambhara and Wang, Beilun and Qi, Yanjun},
booktitle={PACIFIC SYMPOSIUM ON BIOCOMPUTING 2017},
pages={254--265},
year={2017},
organization={World Scientific}
}
Having trouble with our tools? Please contact Jack and we’ll help you sort it out.
10 Jun 2017
Abstract:
Motivation: Histone modifications are among the most important factors that control gene regulation. Computational methods that predict gene expression from histone modification signals are highly desirable for understanding their combinatorial effects in gene regulation. This knowledge can help in developing ‘epigenetic drugs’ for diseases like cancer. Previous studies for quantifying the relationship between histone modifications and gene expression levels either failed to capture combinatorial effects or relied on multiple methods that separate predictions and combinatorial analysis. This paper develops a unified discriminative framework using a deep convolutional neural network to classify gene expression using histone modification data as input. Our system, called DeepChrome, allows automatic extraction of complex interactions among important features. To simultaneously visualize the combinatorial interactions among histone modifications, we propose a novel optimization-based technique that generates feature pattern maps from the learnt deep model. This provides an intuitive description of underlying epigenetic mechanisms that regulate genes. Results: We show that DeepChrome outperforms state-of-the-art models like Support Vector Machines and Random Forests for gene expression classification task on 56 different cell-types from REMC database. The output of our visualization technique not only validates the previous observations but also allows novel insights about combinatorial interactions among histone modification marks, some of which have recently been observed by experimental studies.
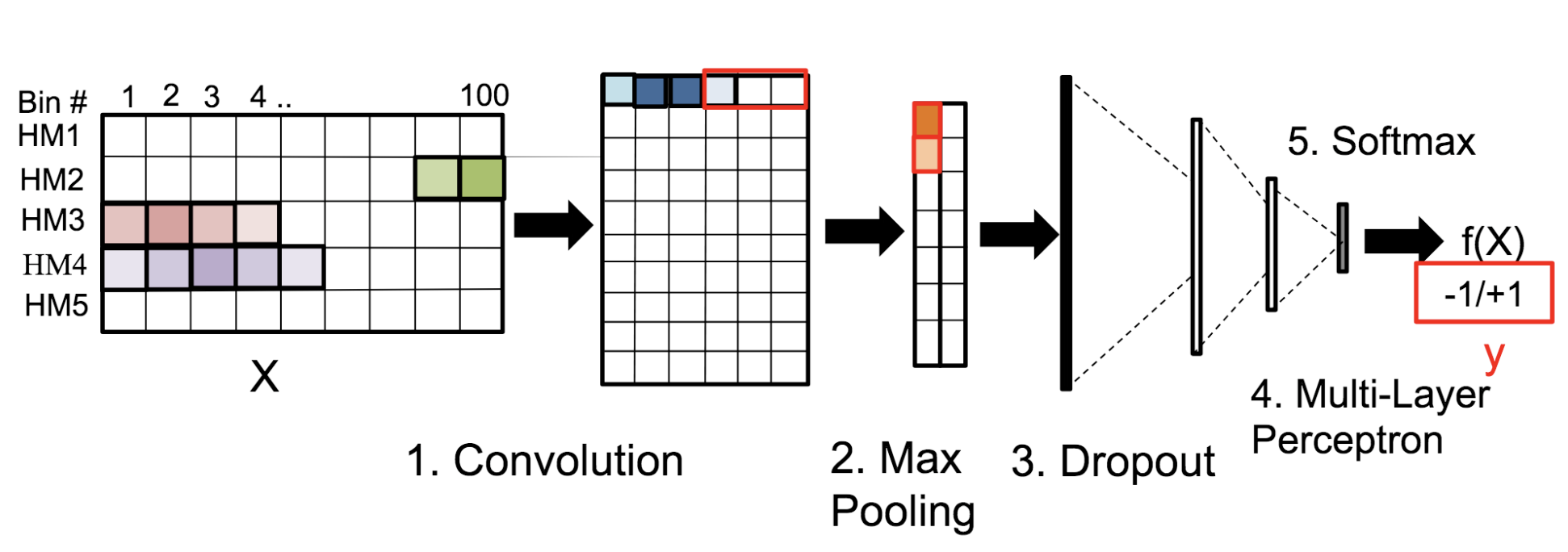
Citations
@article{singh2016deepchrome,
title={DeepChrome: deep-learning for predicting gene expression from histone modifications},
author={Singh, Ritambhara and Lanchantin, Jack and Robins, Gabriel and Qi, Yanjun},
journal={Bioinformatics},
volume={32},
number={17},
pages={i639--i648},
year={2016},
publisher={Oxford University Press}
}
Having trouble with our tools? Please contact Rita and we’ll help you sort it out.
11 Jun 2015
Abstract
Predicting protein properties such as solvent accessibility and secondary structure from its primary amino acid sequence is an important task in bioinformatics. Recently, a few deep learning models have surpassed the traditional window based multilayer perceptron. Taking inspiration from the image classification domain we propose a deep convolutional neural network architecture, MUST-CNN, to predict protein properties. This architecture uses a novel multilayer shift-and-stitch (MUST) technique to generate fully dense per-position predictions on protein sequences. Our model is significantly simpler than the state-of-the-art, yet achieves better results. By combining MUST and the efficient convolution operation, we can consider far more parameters while retaining very fast prediction speeds. We beat the state-of-the-art performance on two large protein property prediction datasets.
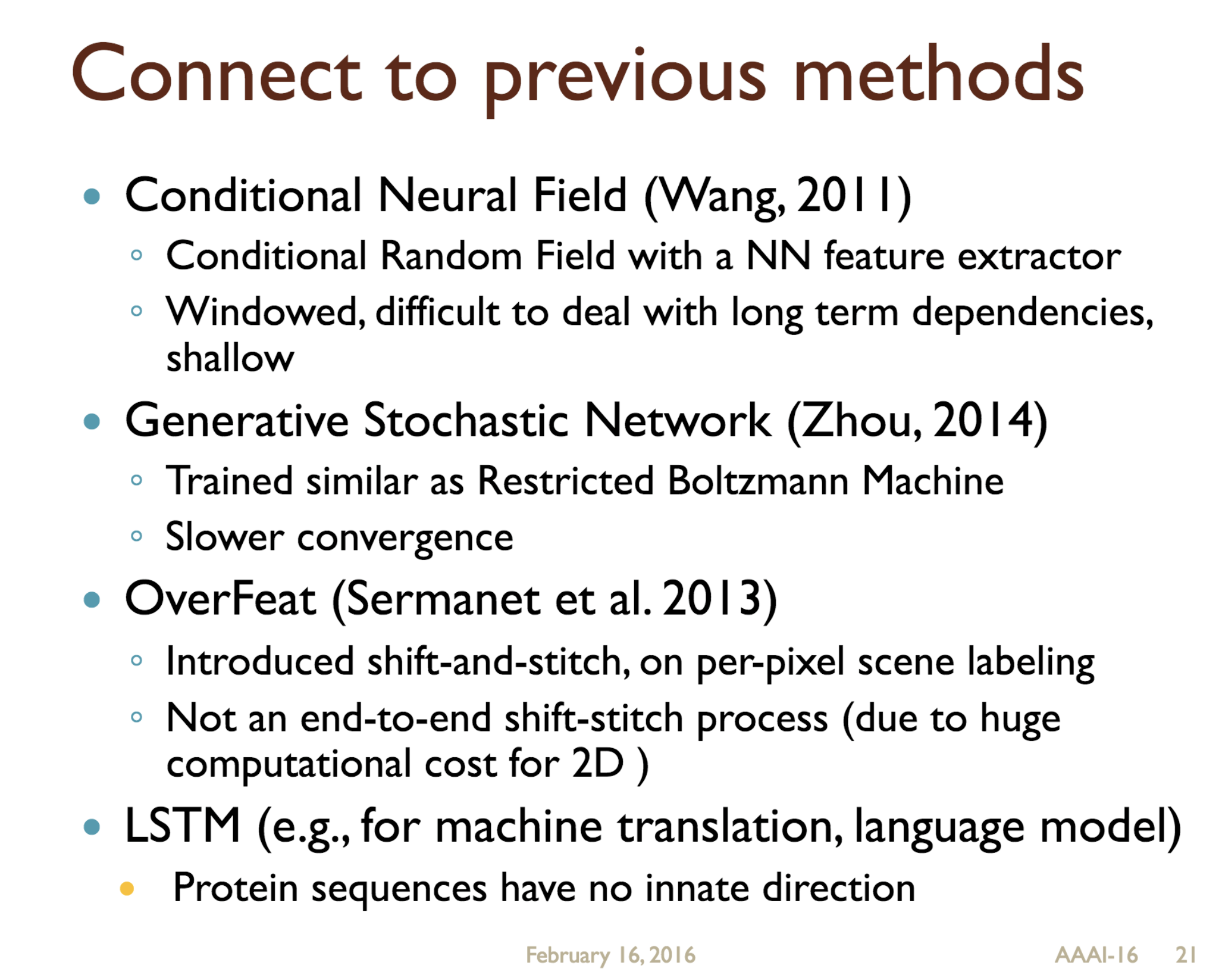
Citations
@inproceedings{lin2016must,
title={MUST-CNN: a multilayer shift-and-stitch deep convolutional architecture for sequence-based protein structure prediction},
author={Lin, Zeming and Lanchantin, Jack and Qi, Yanjun},
booktitle={Proceedings of the Thirtieth AAAI Conference on Artificial Intelligence},
pages={27--34},
year={2016},
organization={AAAI Press}
}
Having trouble with our tools? Please contact Jack and we’ll help you sort it out.
12 Jan 2015
Abstract
A variety of functionally important protein properties, such as secondary structure, transmembrane topology and solvent accessibility, can be encoded as a labeling of amino acids. Indeed, the prediction of such properties from the primary amino acid sequence is one of the core projects of computational biology. Accordingly, a panoply of approaches have been developed for predicting such properties; however, most such approaches focus on solving a single task at a time. Motivated by recent, successful work in natural language processing, we propose to use multitask learning to train a single, joint model that exploits the dependencies among these various labeling tasks. We describe a deep neural network architecture that, given a protein sequence, outputs a host of predicted local properties, including secondary structure, solvent accessibility, transmembrane topology, signal peptides and DNA-binding residues. The network is trained jointly on all these tasks in a supervised fashion, augmented with a novel form of semi-supervised learning in which the model is trained to distinguish between local patterns from natural and synthetic protein sequences. The task-independent architecture of the network obviates the need for task-specific feature engineering. We demonstrate that, for all of the tasks that we considered, our approach leads to statistically significant improvements in performance, relative to a single task neural network approach, and that the resulting model achieves state-of-the-art performance.
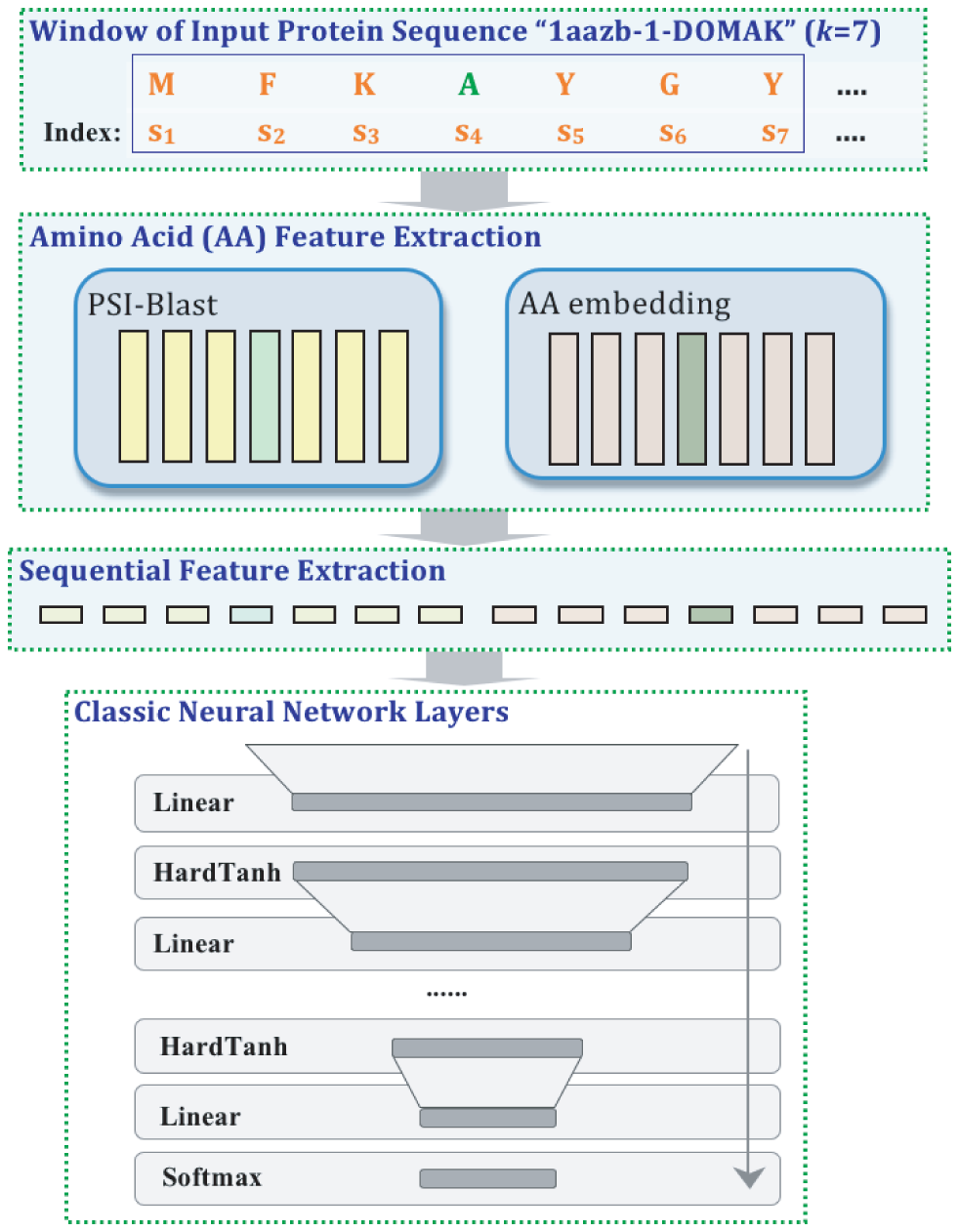
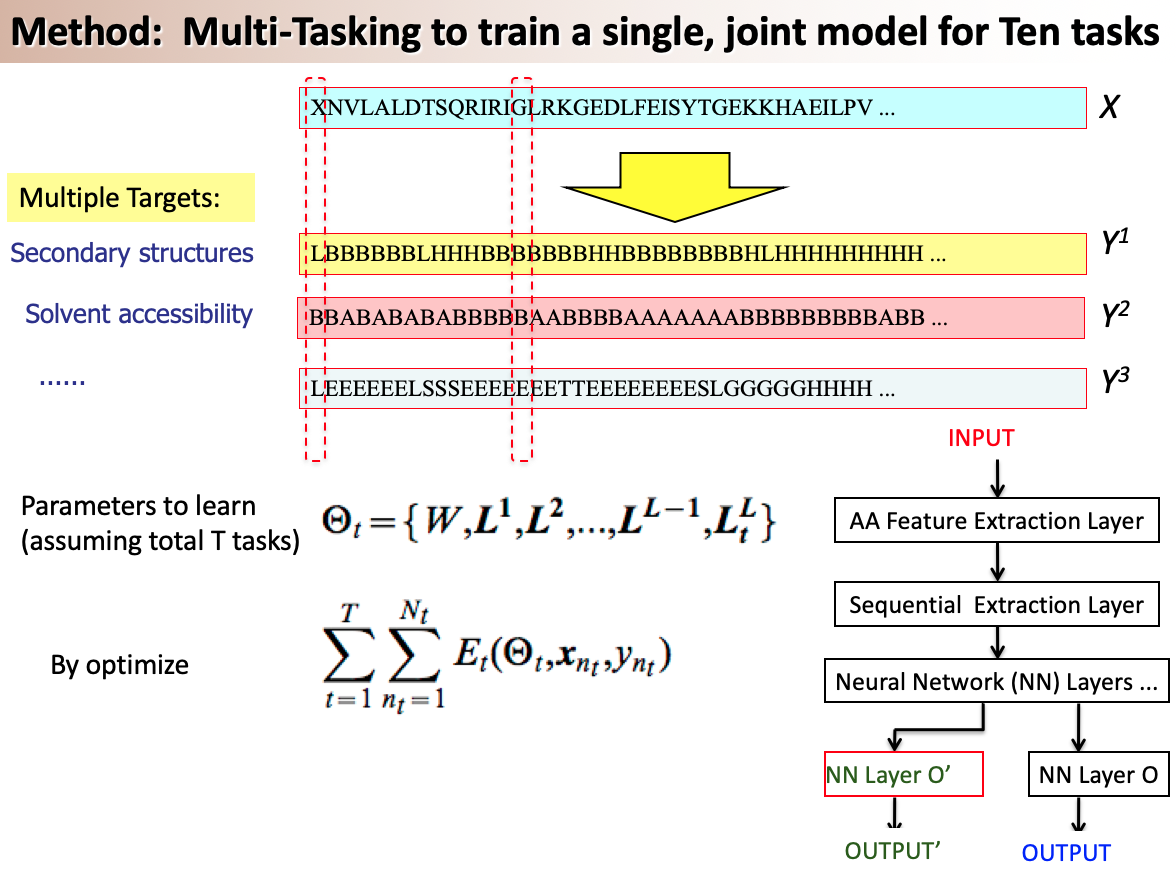
Citations
@article{qi12plosone,
author = {Qi, , Yanjun AND Oja, , Merja AND Weston, , Jason AND Noble, , William Stafford},
journal = {PLoS ONE},
publisher = {Public Library of Science},
title = {A Unified Multitask Architecture for Predicting Local Protein Properties},
year = {2012},
month = {03},
volume = {7},
url = {http://dx.doi.org/10.1371%2Fjournal.pone.0032235},
pages = {e32235},
number = {3},
doi = {10.1371/journal.pone.0032235}
}
Having trouble with our tools? Please contact Jack and we’ll help you sort it out.
01 Oct 2013
- authors: Yanjun Qi, Sujatha Das, Ronan Collobert, Jason Weston
Supplementary Here
Abstract
In this paper we introduce a deep neural network architecture to perform information extraction on character-based sequences,
e.g. named-entity recognition on Chinese text or secondary-structure detection on protein sequences. With a task-independent architecture, the
deep network relies only on simple character-based features, which obviates the need for task-specific feature engineering. The proposed discriminative framework includes three important strategies, (1) a deep
learning module mapping characters to vector representations is included
to capture the semantic relationship between characters; (2) abundant
online sequences (unlabeled) are utilized to improve the vector representation through semi-supervised learning; and (3) the constraints of
spatial dependency among output labels are modeled explicitly in the
deep architecture. The experiments on four benchmark datasets have
demonstrated that, the proposed architecture consistently leads to the
state-of-the-art performance.
Citations
@inproceedings{qi2014deep,
title={Deep learning for character-based information extraction},
author={Qi, Yanjun and Das, Sujatha G and Collobert, Ronan and Weston, Jason},
booktitle={European Conference on Information Retrieval},
pages={668--674},
year={2014},
organization={Springer}
}
Having trouble with our tools? Please contact Yanjun Qi and we’ll help you sort it out.
12 Jan 2013
Abstract
A variety of functionally important protein properties, such as secondary structure, transmembrane topology and solvent accessibility, can be encoded as a labeling of amino acids. Indeed, the prediction of such properties from the primary amino acid sequence is one of the core projects of computational biology. Accordingly, a panoply of approaches have been developed for predicting such properties; however, most such approaches focus on solving a single task at a time. Motivated by recent, successful work in natural language processing, we propose to use multitask learning to train a single, joint model that exploits the dependencies among these various labeling tasks. We describe a deep neural network architecture that, given a protein sequence, outputs a host of predicted local properties, including secondary structure, solvent accessibility, transmembrane topology, signal peptides and DNA-binding residues. The network is trained jointly on all these tasks in a supervised fashion, augmented with a novel form of semi-supervised learning in which the model is trained to distinguish between local patterns from natural and synthetic protein sequences. The task-independent architecture of the network obviates the need for task-specific feature engineering. We demonstrate that, for all of the tasks that we considered, our approach leads to statistically significant improvements in performance, relative to a single task neural network approach, and that the resulting model achieves state-of-the-art performance.
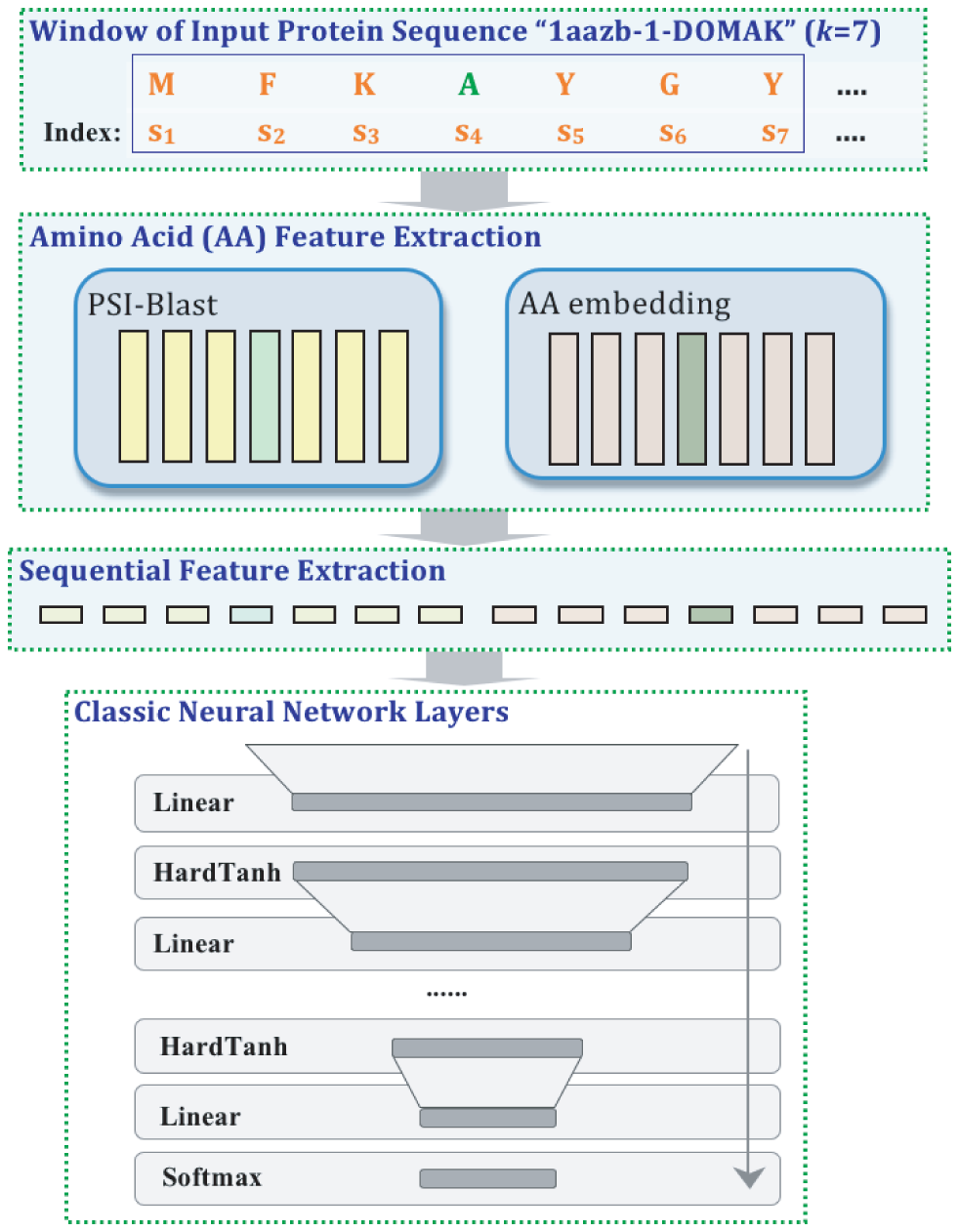
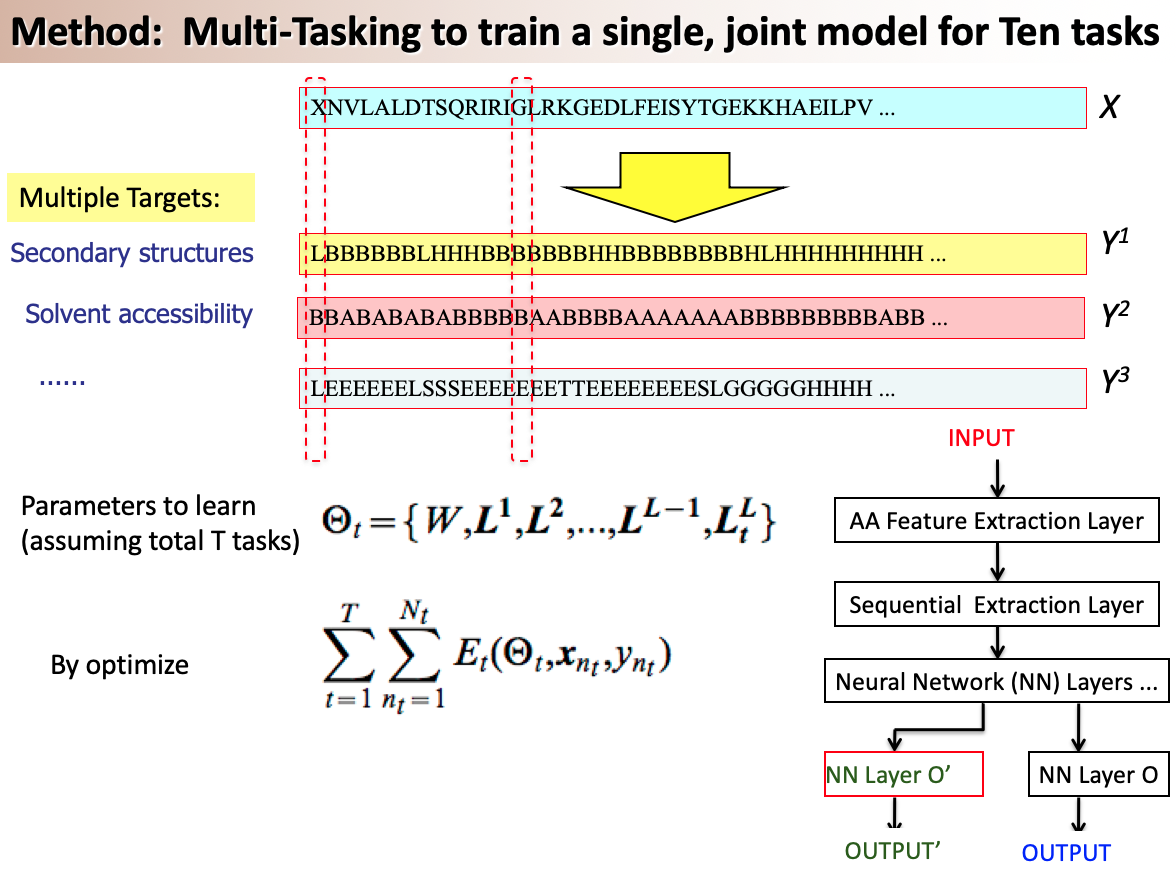
Citations
@article{qi12plosone,
author = {Qi, , Yanjun AND Oja, , Merja AND Weston, , Jason AND Noble, , William Stafford},
journal = {PLoS ONE},
publisher = {Public Library of Science},
title = {A Unified Multitask Architecture for Predicting Local Protein Properties},
year = {2012},
month = {03},
volume = {7},
url = {http://dx.doi.org/10.1371%2Fjournal.pone.0032235},
pages = {e32235},
number = {3},
doi = {10.1371/journal.pone.0032235}
}
Having trouble with our tools? Please contact Jack and we’ll help you sort it out.
01 Mar 2010
Title: Systems and methods for semi-supervised relationship extraction
- authors: Qi, Yanjun and Bai, Bing and Ning, Xia and Kuksa, Pavel
- PDF
-
Talk: Slide
- Abstract
Bio-relation extraction (bRE), an important goal in bio-text mining, involves subtasks identifying relationships between bio-entities in text at multiple levels, e.g., at the article, sentence or relation level. A key limitation of current bRE systems is that they are restricted by the availability of annotated corpora. In this work we introduce a semi-supervised approach that can tackle multi-level bRE via string comparisons with mismatches in the string kernel framework. Our string kernel implements an abstraction step, which groups similar words to generate more abstract entities, which can be learnt with unlabeled data. Specifically, two unsupervised models are proposed to capture contextual (local or global) semantic similarities between words from a large unannotated corpus. This Abstraction-augmented String Kernel (ASK) allows for better generalization of patterns learned from annotated data and provides a unified framework for solving bRE with multiple degrees
of detail. ASK shows effective improvements over classic string kernels
on four datasets and achieves state-of-the-art bRE performance without
the need for complex linguistic features.
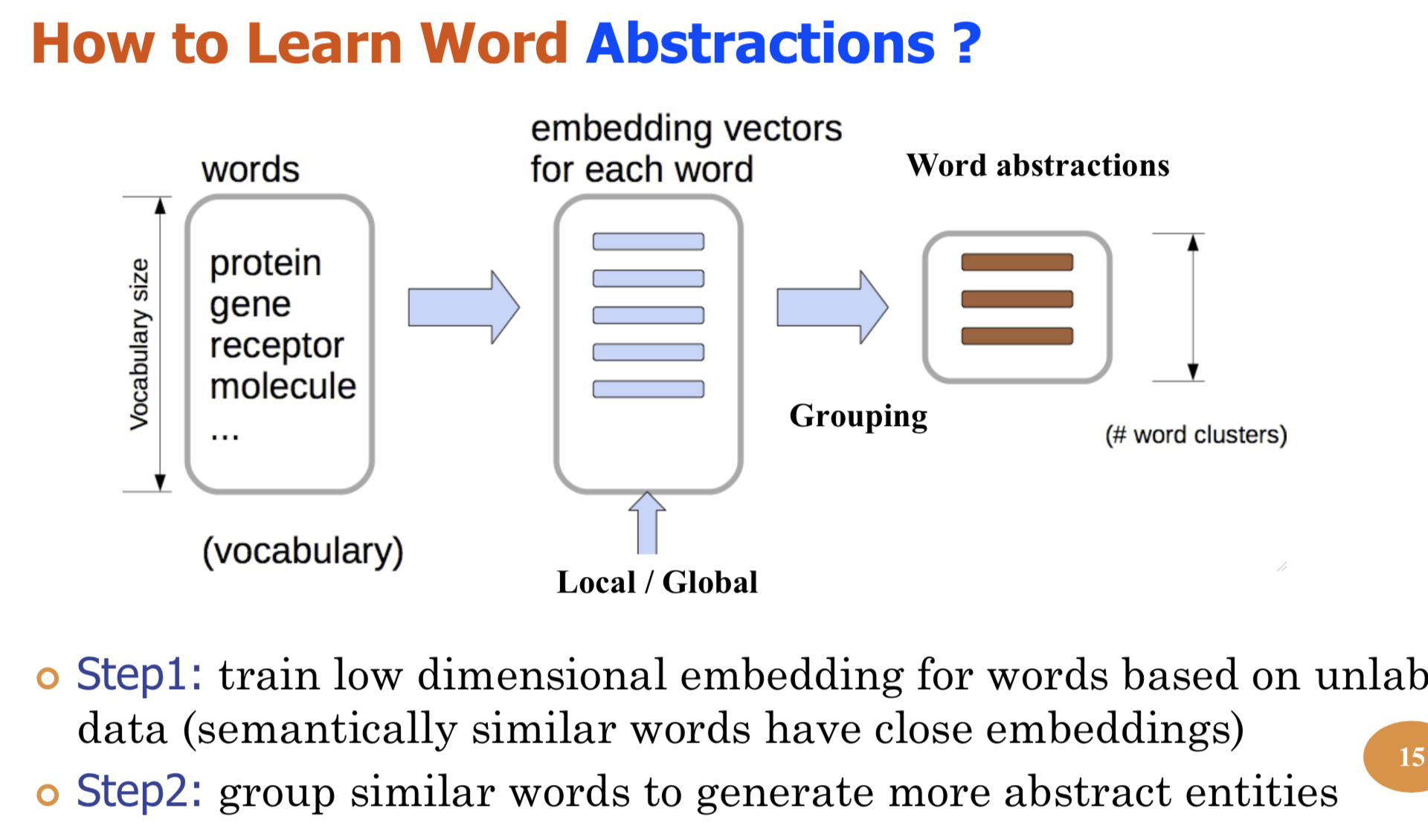
-
PDF
- Talk: Slide
-
URL More
- Abstract
Extracting semantic relations between entities is an important step towards automatic text understanding. In this paper, we propose a novel Semi-supervised Convolution Graph Kernel (SCGK) method for semantic Relation Extraction (RE) from natural language. By encoding English sentences as dependence graphs among words, SCGK computes kernels (similarities) between sentences using a convolution strategy, i.e., calculating similarities over all possible short single paths from two dependence graphs. Furthermore, SCGK adds three semi-supervised strategies in the kernel calculation to incorporate soft-matches between (1) words, (2) grammatical dependencies, and (3) entire sentences, respectively. From a large unannotated corpus, these semi-supervision steps learn to capture contextual semantic patterns of elements in natural sentences, which therefore alleviate the lack of annotated examples in most RE corpora. Through convolutions and multi-level semi-supervisions, SCGK provides a powerful model to encode both syntactic and semantic evidence existing in natural English sentences, which effectively recovers the target relational patterns of interest. We perform extensive experiments on five RE benchmark datasets which aim to identify interaction relations from biomedical literature. Our results demonstrate that SCGK achieves the state-of-the-art performance on the task of semantic relation extraction.
Paper3: Semi-Supervised Bio-Named Entity Recognition with Word-Codebook Learning
- Pavel P. Kuksa, Yanjun Qi,
-
PDF
- Abstract
We describe a novel semi-supervised method called WordCodebook Learning (WCL), and apply it to the task of bionamed entity recognition (bioNER). Typical bioNER systems can be seen as tasks of assigning labels to words in bioliterature text. To improve supervised tagging, WCL learns
a class of word-level feature embeddings to capture word
semantic meanings or word label patterns from a large unlabeled corpus. Words are then clustered according to their
embedding vectors through a vector quantization step, where
each word is assigned into one of the codewords in a codebook. Finally codewords are treated as new word attributes
and are added for entity labeling. Two types of wordcodebook learning are proposed: (1) General WCL, where
an unsupervised method uses contextual semantic similarity of words to learn accurate word representations; (2)
Task-oriented WCL, where for every word a semi-supervised
method learns target-class label patterns from unlabeled
data using supervised signals from trained bioNER model.
Without the need for complex linguistic features, we demonstrate utility of WCL on the BioCreativeII gene name recognition competition data, where WCL yields state-of-the-art
performance and shows great improvements over supervised
baselines and semi-supervised counter peers.
Citations
@INPROCEEDINGS{ecml2010ask,
author = {Pavel P. Kuksa and Yanjun Qi and Bing Bai and Ronan Collobert and
Jason Weston and Vladimir Pavlovic and Xia Ning},
title = {Semi-Supervised Abstraction-Augmented String Kernel for Multi-Level
Bio-Relation Extraction},
booktitle = {ECML},
year = {2010},
note = {Acceptance rate: 106/658 (16%)},
bib2html_pubtype = {Refereed Conference},
}
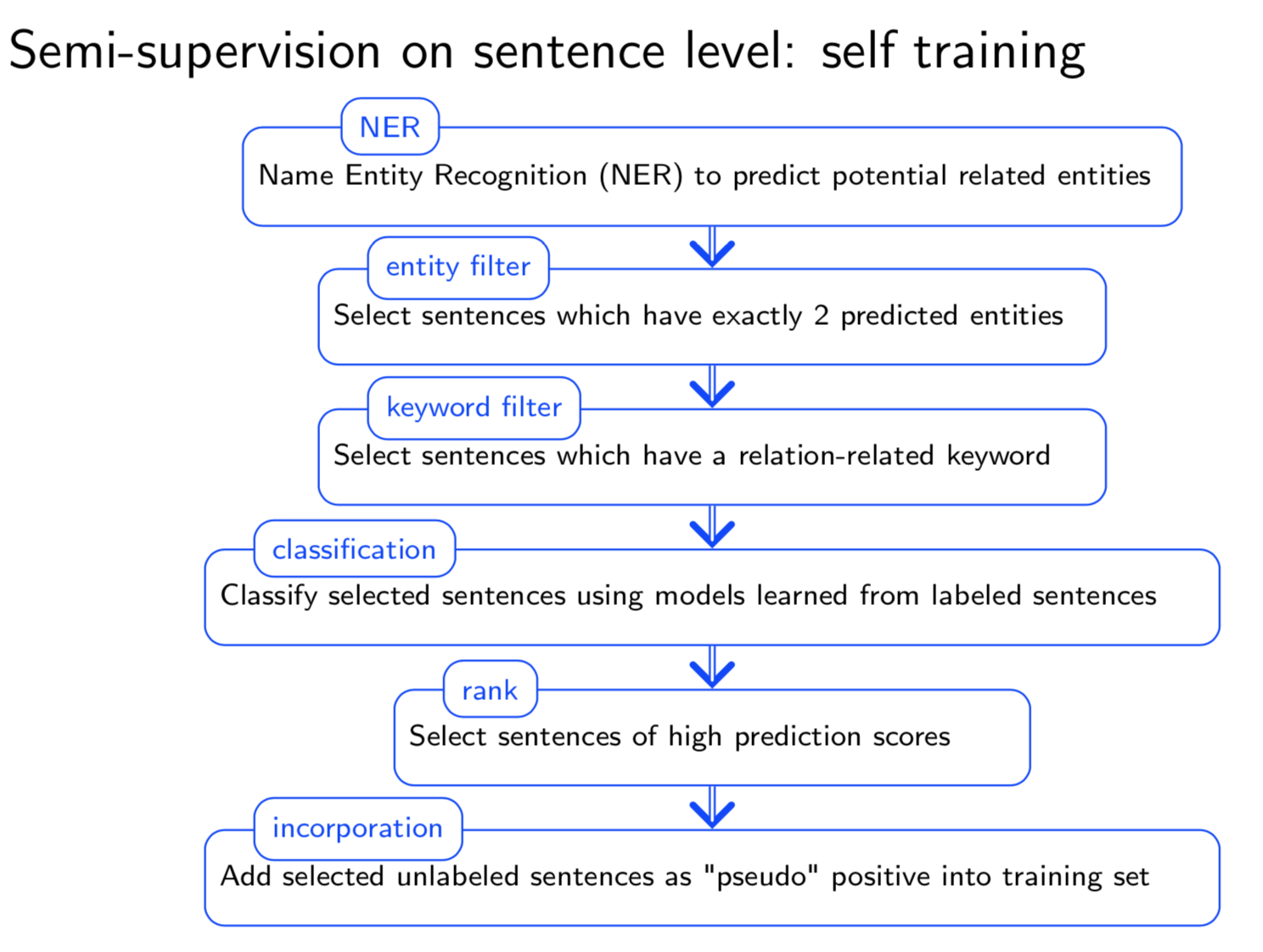
Having trouble with our tools? Please contact Yanjun Qi and we’ll help you sort it out.
01 Feb 2009
Title: Semi-supervised multi-task learning for predicting interactions between HIV-1 and human proteins
- authors: Yanjun Qi, Oznur Tastan, Jaime G. Carbonell, Judith Klein-Seetharaman, Jason Weston
Abstract
-
Motivation: Protein–protein interactions (PPIs) are critical for virtually every biological function. Recently, researchers suggested to use supervised learning for the task of classifying pairs of proteins as interacting or not. However, its performance is largely restricted by the availability of truly interacting proteins (labeled). Meanwhile, there exists a considerable amount of protein pairs where an association appears between two partners, but not enough experimental evidence to support it as a direct interaction (partially labeled).
-
Results: We propose a semi-supervised multi-task framework for predicting PPIs from not only labeled, but also partially labeled reference sets. The basic idea is to perform multi-task learning on a supervised classification task and a semi-supervised auxiliary task. The supervised classifier trains a multi-layer perceptron network for PPI predictions from labeled examples. The semi-supervised auxiliary task shares network layers of the supervised classifier and trains with partially labeled examples. Semi-supervision could be utilized in multiple ways. We tried three approaches in this article, (i) classification (to distinguish partial positives with negatives); (ii) ranking (to rate partial positive more likely than negatives); (iii) embedding (to make data clusters get similar labels). We applied this framework to improve the identification of interacting pairs between HIV-1 and human proteins. Our method improved upon the state-of-the-art method for this task indicating the benefits of semi-supervised multi-task learning using auxiliary information.
Citations
@article{qi2010semi,
title={Semi-supervised multi-task learning for predicting interactions between HIV-1 and human proteins},
author={Qi, Yanjun and Tastan, Oznur and Carbonell, Jaime G and Klein-Seetharaman, Judith and Weston, Jason},
journal={Bioinformatics},
volume={26},
number={18},
pages={i645--i652},
year={2010},
publisher={Oxford University Press}
}
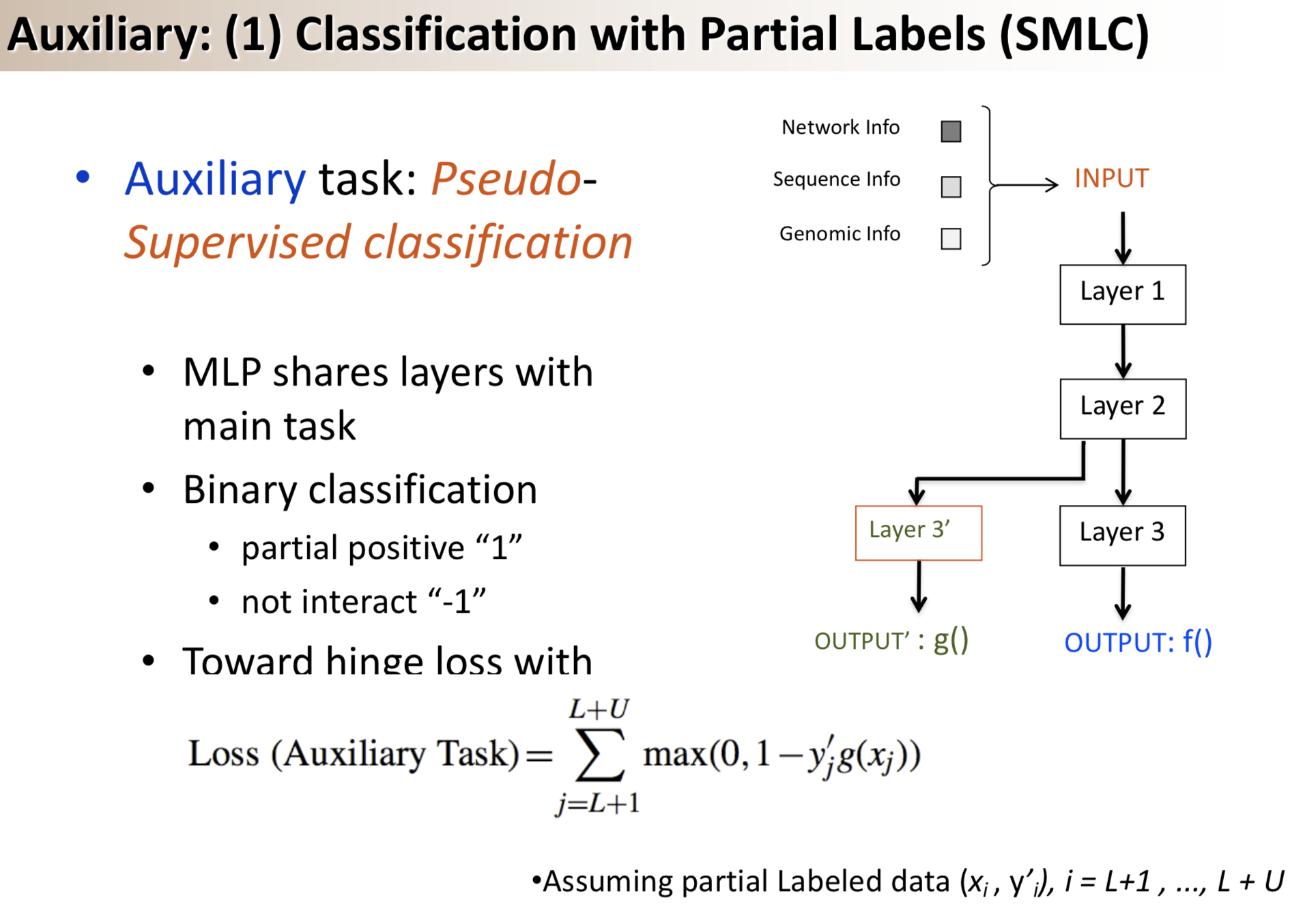
Having trouble with our tools? Please contact Yanjun Qi and we’ll help you sort it out.